在Kaggle最近结束的Swag比赛Binary Prediction of Poisonous Mushrooms中,前五的方案中,很多团队都是用到了端到端的自动建模库Autogluon,第一名和第三名的方案中都是以其为核心进行的。
例如第三名的方案框架如下:
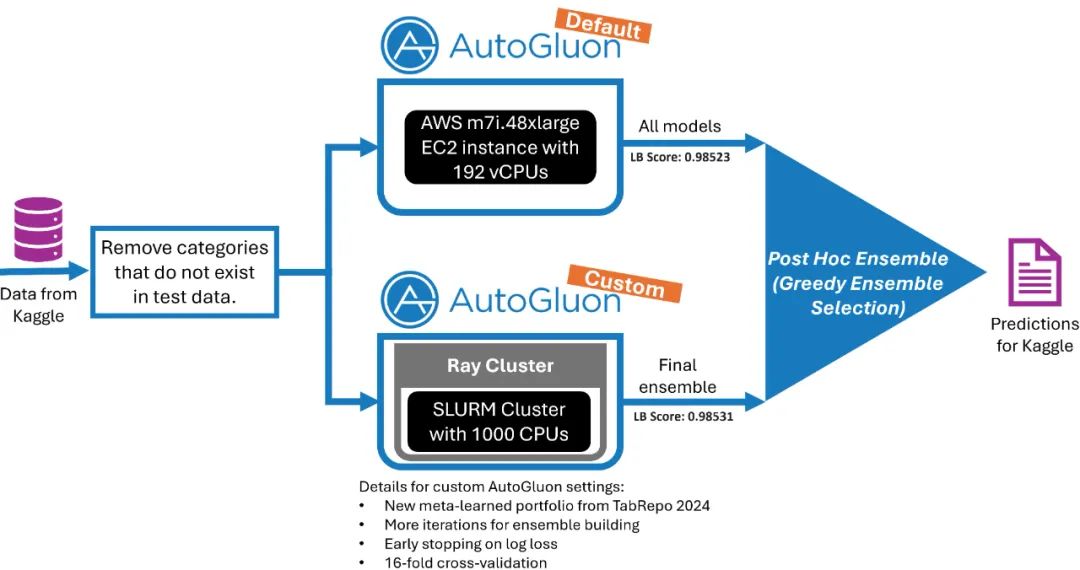
- 一个自定义版AutoGluon。对于自定义版,我们使用了以下设置:a.我们使用对数损失作为早停指标,同时根据目标指标MCC进行优化和选择。我们不确定这是否有帮助,但它改善了我们的离线CV得分。b.我们使用了16折交叉验证和AutoGluon的多层堆叠实现。c.我们训练了一个定制模型组合,通过TabRepo进行元学习(即zero-shot HPO)。d.我们进行了100次迭代(而不是默认的25次)用于 post hoc集成。
所以本文我们简单学习一下Autogluon。
关于Autogluon:是一个端到端的自动机器学习框架,只需几行代码,就可以在图像、文本、时间序列和表格数据上训练和部署高精度的机器学习和深度学习模型。
可以使用的场景
1.表格问题
# pip install autogluon
from autogluon.tabular import TabularPredictor
predictor = TabularPredictor(label="class").fit("train.csv")
predictions = predictor.predict("test.csv")
2.时序问题
# 详细案例参见:https://towardsdatascience.com/autogluon-timeseries-every-time-series-forecasting-model-in-one-library-29a3bf6879db
import pandas as pd
import numpy as np
import matplotlib.pyplot as plt
import os
import subprocess
from gluonts.dataset.repository import get_dataset, dataset_names
from gluonts.dataset.util import to_pandas
from gluonts.evaluation.metrics import mse
from autogluon.timeseries import TimeSeriesDataFrame, TimeSeriesPredictor
train_data = TimeSeriesDataFrame.from_data_frame(
train,
id_column="item_id",
timestamp_column="start"
)
test_data = TimeSeriesDataFrame.from_data_frame(
test,
id_column="item_id",
timestamp_column="start"
)
multiple_timeseries_path = "multiple-timeseries"
model_path_fast = "tourism-quarterly-fast"
path = os.path.join(multiple_timeseries_path, model_path_fast)
predictor = TimeSeriesPredictor(
prediction_length=24,
#path = "multiple_timeseries_path/tourism-quarterly-fast"
path= path,
target="target",
eval_metric="MSE"
)
predictor.fit(
train_data,
presets="fast_training",
random_seed=42)
Autogluon目前在Kaggle的诸多比赛中是非常受欢迎的一个自动化端到端的建模工具,非常建议大家在参加相关竞赛时尝试使用。
- https://towardsdatascience.com/autogluon-timeseries-every-time-series-forecasting-model-in-one-library-29a3bf6879db
- https://github.com/autogluon/autogluon
- https://www.kaggle.com/competitions/playground-series-s4e8/discussion/523656
请备注:”昵称-学校/公司-研究方向“,例如:”张小明-浙大-CV“加群。
(也可以加入机器学习交流qq群772479961)
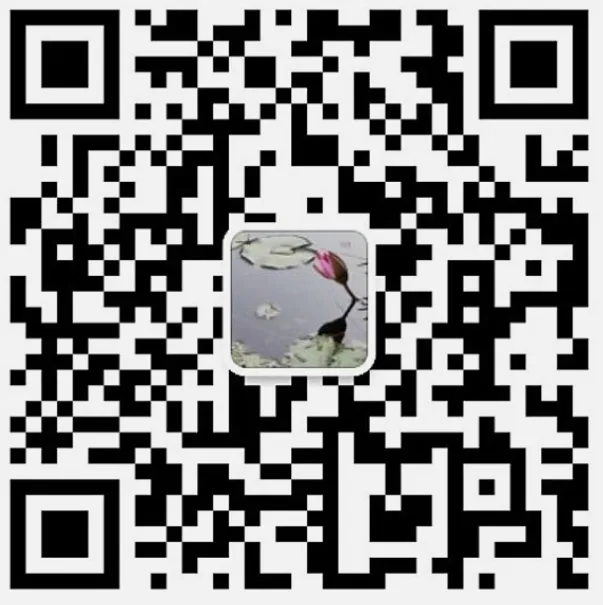