scikit-learn (sklearn)
是Python环境下常见的机器学习库,包含了常见的分类、回归和聚类算法。在训练模型之后,常见的操作是对模型进行可视化,则需要使用Matplotlib
进行展示。
scikit-plot
是一个基于sklearn
和Matplotlib
的库,主要的功能是对训练好的模型进行可视化,功能比较简单易懂。
https://scikit-plot.readthedocs.io
pip install scikit-plot
功能1:评估指标可视化
scikitplot.metrics.plot_confusion_matrix
快速展示模型预测结果和标签计算得到的混淆矩阵。
import scikitplot as skplt
rf = RandomForestClassifier()
rf = rf.fit(X_train, y_train)
y_pred = rf.predict(X_test)
skplt.metrics.plot_confusion_matrix(y_test, y_pred, normalize=True)
plt.show()
scikitplot.metrics.plot_roc
快速展示模型预测的每个类别的ROC曲线。
import scikitplot as skplt
nb = GaussianNB()
nb = nb.fit(X_train, y_train)
y_probas = nb.predict_proba(X_test)
skplt.metrics.plot_roc(y_test, y_probas)
plt.show()
scikitplot.metrics.plot_ks_statistic
从标签和分数/概率生成 KS 统计图。
import scikitplot as skplt
lr = LogisticRegression()
lr = lr.fit(X_train, y_train)
y_probas = lr.predict_proba(X_test)
skplt.metrics.plot_ks_statistic(y_test, y_probas)
plt.show()
scikitplot.metrics.plot_precision_recall
从标签和概率生成PR曲线
import
scikitplot as skplt
nb = GaussianNB()
nb.fit(X_train, y_train)
y_probas = nb.predict_proba(X_test)
skplt.metrics.plot_precision_recall(y_test, y_probas)
plt.show()
scikitplot.metrics.plot_silhouette
对聚类结果进行silhouette analysis分析
import scikitplot as skplt
kmeans = KMeans(n_clusters=4, random_state=1)
cluster_labels = kmeans.fit_predict(X)
skplt.metrics.plot_silhouette(X, cluster_labels)
plt.show()
scikitplot.metrics.plot_calibration_curve
绘制分类器的矫正曲线
import scikitplot as skplt
rf = RandomForestClassifier()
lr = LogisticRegression()
nb = GaussianNB()
svm = LinearSVC()
rf_probas = rf.fit(X_train, y_train).predict_proba(X_test)
lr_probas = lr.fit(X_train, y_train).predict_proba(X_test)
nb_probas = nb.fit(X_train, y_train).predict_proba(X_test)
svm_scores = svm.fit(X_train, y_train).decision_function(X_test)
probas_list = [rf_probas, lr_probas, nb_probas, svm_scores]
clf_names = ['Random Forest', 'Logistic Regression',
'Gaussian Naive Bayes', 'Support Vector Machine']
skplt.metrics.plot_calibration_curve(y_test,
probas_list,
clf_names)
plt.show()
功能2:模型可视化
scikitplot.estimators.plot_learning_curve
生成不同训练样本下的训练和测试学习曲线图。
import scikitplot as skplt
rf = RandomForestClassifier()
skplt.estimators.plot_learning_curve(rf, X, y)
plt.show()
scikitplot.estimators.plot_feature_importances
可视化特征重要性。
import scikitplot as skplt
rf = RandomForestClassifier()
rf.fit(X, y)
skplt.estimators.plot_feature_importances(
rf, feature_names=['petal length', 'petal width',
'sepal length', 'sepal width'])
plt.show()
功能3:聚类可视化
scikitplot.cluster.plot_elbow_curve
展示聚类的肘步图。
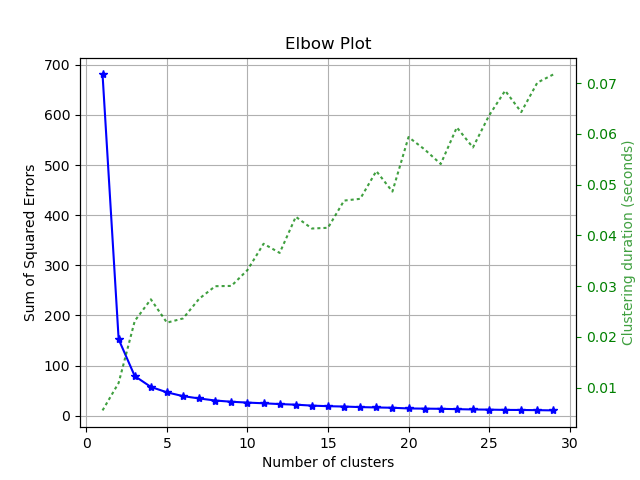
import scikitplot as skplt
kmeans = KMeans(random_state=1)
skplt.cluster.plot_elbow_curve(kmeans, cluster_ranges=range(1, 30))
plt.show()
功能4:降维可视化
scikitplot.decomposition.plot_pca_component_variance
绘制 PCA 分量的解释方差比。
import scikitplot as skplt
pca = PCA(random_state=1)
pca.fit(X)
skplt.decomposition.plot_pca_component_variance(pca)
>plt.show()
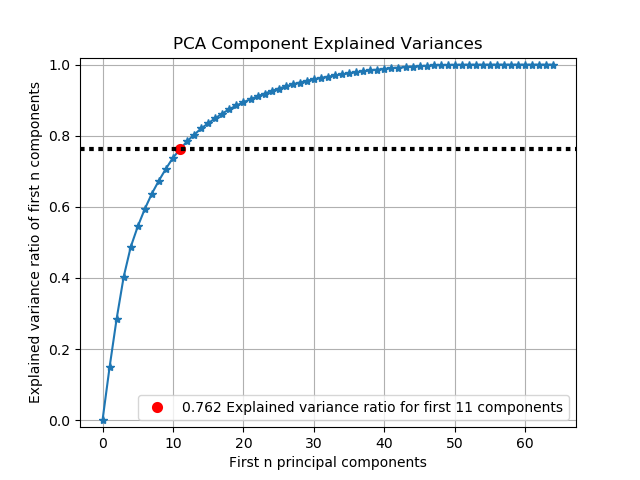
scikitplot.decomposition.plot_pca_2d_projection
绘制PCA降维之后的散点图。
import scikitplot as skplt
pca = PCA(random_state=1)
pca.fit(X)
skplt.decomposition.plot_pca_2d_projection(pca, X, y)
plt.show()