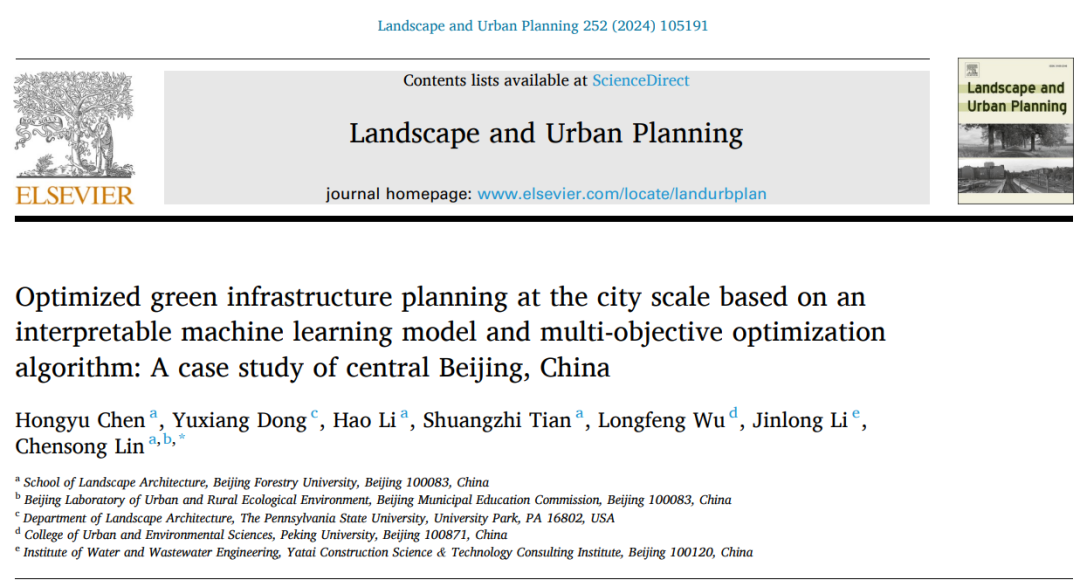
题目:Optimized green infrastructure planning at the city scale based on an
interpretable machine learning model and multi-objective optimization
algorithm: A case study of central Beijing, China期刊:Landscape and Urban PlanningDOI:https://doi.org/10.1016/j.landurbplan.2024.105191简述:这篇文章是关于城市尺度绿色基础设施(GI)规划的优化研究,以中国北京中心区作为案例。研究提出了一个基于可解释的机器学习模型(支持向量机,SVM)和多目标优化算法(非支配排序遗传算法II,NSGA-II)的GI定量规划模型。绿色基础设施(Green Infrastructure, GI)已发展成为缓解城市洪水的一种可持续方法。尽管机器学习(Machine Learning, ML)模型在城市洪水模拟方面展现出优势,但它们在支持城市尺度GI定量规划方面的直接应用仍然是一个挑战。为了解决这个问题,本研究将基于支持向量机(Support Vector Machine, SVM)的可解释ML模型和Shapley加性解释(SHAP)方法与非支配排序遗传算法II(Non-dominated Sorting Genetic Algorithm-II, NSGA-II)相结合。该模型应用于中国北京中心地区的案例研究,并展示了出色的性能,曲线下面积(Area Under Curve, AUC)值高达0.94。城市洪水易感性评估的结果表明,研究区域中的城乡过渡区面临着更大的洪水威胁。通过SHAP模型解释,揭示了GI和灰色基础设施(Grey Infrastructure, GrI)在防洪中的主导作用,并证明了在GI比例低于0.45的研究单元中,两者之间的非线性互补性更为显著。在NSGA-II优化框架的支持下,实现了不同GI总实施量下的最优GI计划,其中选择了一个GI总面积增加了3.21%的解决方案,作为投资效率最高的方案。模型建议GI实施的模式应该是分散和小规模的。本研究提供了一个具有广泛应用前景的工具,有效地将GI实施与城市规划整合起来。本研究的发现不仅为确定北京新生态空间的优先区域提供了重要参考,而且为与北京具有相似特征的地区提供了GI规划和城市洪水管理的新见解。
Green infrastructure (GI) has developed as a sustainable approach to the mitigation of urban floods. While machine learning (ML) models have exhibited advantages in urban flood simulation, their direct application to support the quantitative planning of GI at the city scale remains a challenge. To address this, an interpretable ML model based on support vector machine (SVM) and the Shapley additive explanations (SHAP) approach is integrated with the non-dominated sorting genetic algorithm-II (NSGA-II) in this study. The model is applied to the case of central Beijing, China, and demonstrates a robust performance with a high area under curve (AUC) value of 0.94. The results of the urban flood susceptibility assessment identify the urban-rural transition zone in the study area as being under a greater flood threat. Via model interpretation with SHAP, the dominant roles of GI and grey infrastructure (GrI) in preventing flood are revealed and the non-linear complementarity between the two is demonstrated to be more significant in study units with a GI proportion of less than 0.45. Supported by the NSGA-II-based optimization framework, optimal GI plans under different total implementations of GI are achieved, among which a solution with a 3.21% increase in the total GI area is selected as that with the best investment efficiency. The pattern of GI implementation is suggested to be dispersed and small-scale by model. This study provides a tool with broad application prospects, effectively integrating GI implementation with urban planning. The findings of this study not only provide important references for the determination of the priority areas of new ecological space in Beijing, but also provide areas that share similar characteristics with new insight into GI planning and the management of urban floods.(1)提出了一种城市尺度绿色基础设施定量规划的模型。(3)绿色基础设施的扩展对灰色基础设施的压力有非线性效应。(4)建议采用分散和小规模的绿色基础设施扩展模式。为了支持城市尺度上定量且高效的绿色基础设施(GI)规划,本文探索了一个由可解释的机器学习(ML)模型和多目标优化算法组成的创新模型。近年来一直受到城市洪水困扰的北京中心城市区,被视为中国高度城市化的典型区域,用以验证所提出模型的可用性。具体而言,本研究的主要目标包括:(1) 通过支持向量机(SVM)分析研究区域的洪水易感性及其空间分布,并验证ML模型在具有高空间异质性的城市建设空间中进行洪水模拟的可行性;(2) 引入Shapley可加解释(SHAP)来揭示影响城市洪水的主要因素及其相互作用的定量机制;(3) 使用基于非支配排序遗传算法II(NSGA-II)的优化框架确定GI实施的位置和规模。本研究补充了城市尺度GI规划的现有理论和技术方法,并通过在城市洪水领域使用可解释的ML模型,弥合了现有的研究差距。这一努力还为城市GI规划和城市洪水的预防提供了新的见解。
本研究提出了一种新颖的模型,用于实现城市尺度上绿色基础设施(GI)的定量规划,并通过以北京中心区为案例研究,评估了模型的性能。本研究的主要结论可以总结如下:
1) 基于支持向量机(SVM)和Shapley可加解释(SHAP)的可解释机器学习(ML)模型有效地捕捉了解释因素与建成区洪水之间的非平稳性和非线性相互作用。
2) 北京中心区的洪水易感性表现出显著的极化现象,应加强对城乡过渡区的洪水监测和预警。
3) GI面积的减少将触发排水压力的非线性上升,需要通过灰色基础设施(GrI)来解决,特别是对于那些P_GI值小于0.45的单元。因此,管理GI的规模应被视为城市尺度防洪的核心举措。
4) 建议在研究区域实施分散和小规模的GI模式,以平衡城市洪水管理和土地成本。北京中心区的西南郊区应被视为GI实施的优先区域。
本研究提出的模型能够有效地将GI实施与城市规划整合起来,解决了城市尺度定量GI规划的技术挑战,并展示了广泛的应用潜力。本研究的发现可以为研究区域以及具有相似特征的地区在GI规划和土地管理方面提供重要的见解,并为支持决策者设计有效措施以减少城市洪水奠定基础。未来,应进一步提高模型的准确性及其实际应用能力,例如指导工程系统和适应未来的气候条件。
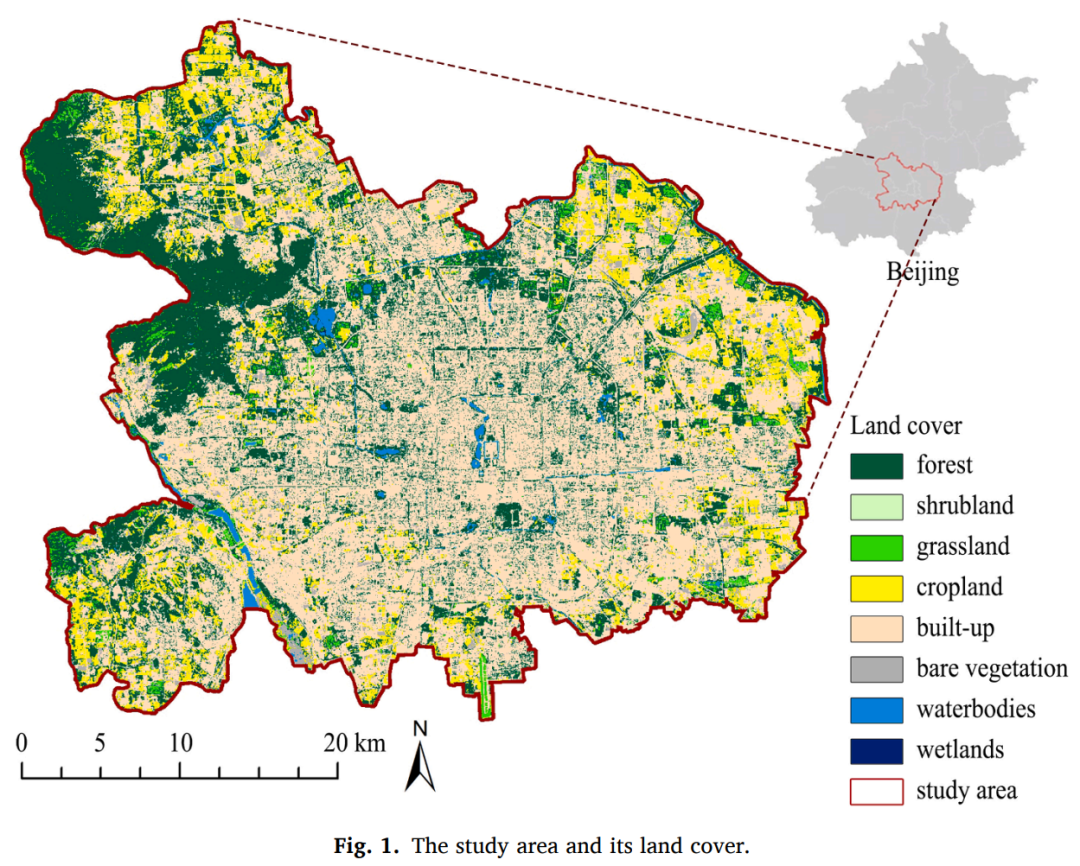
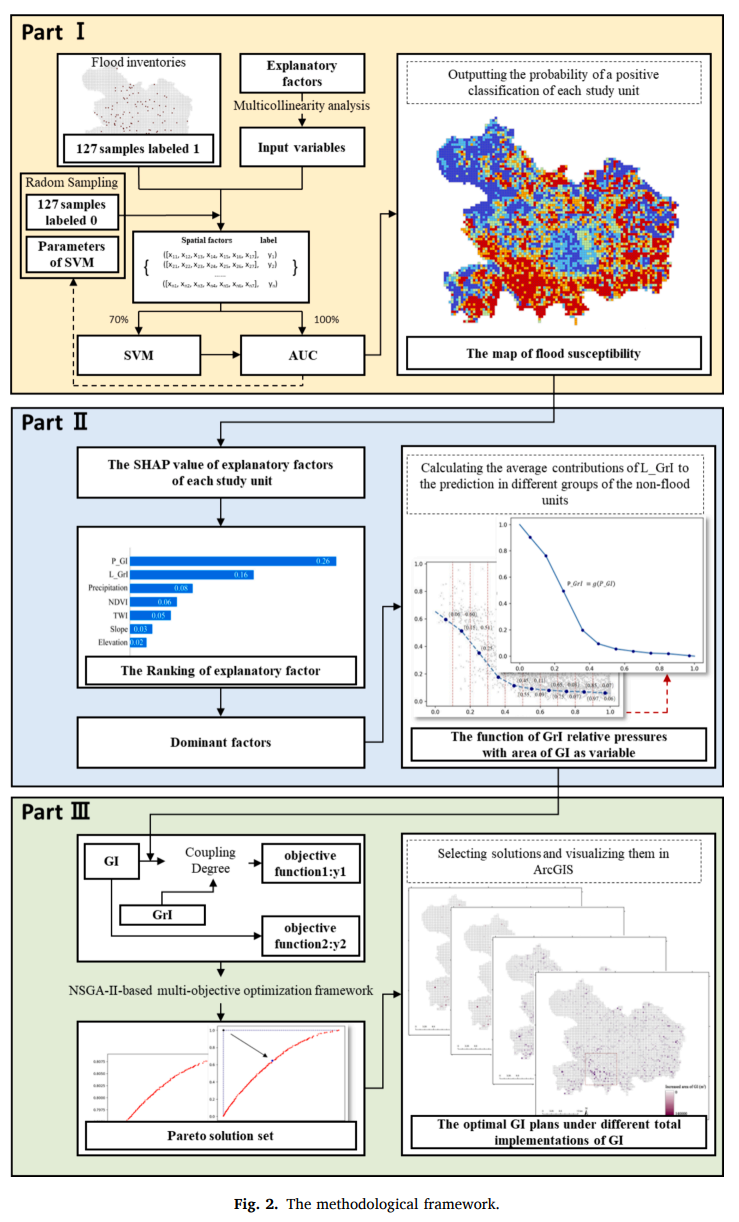
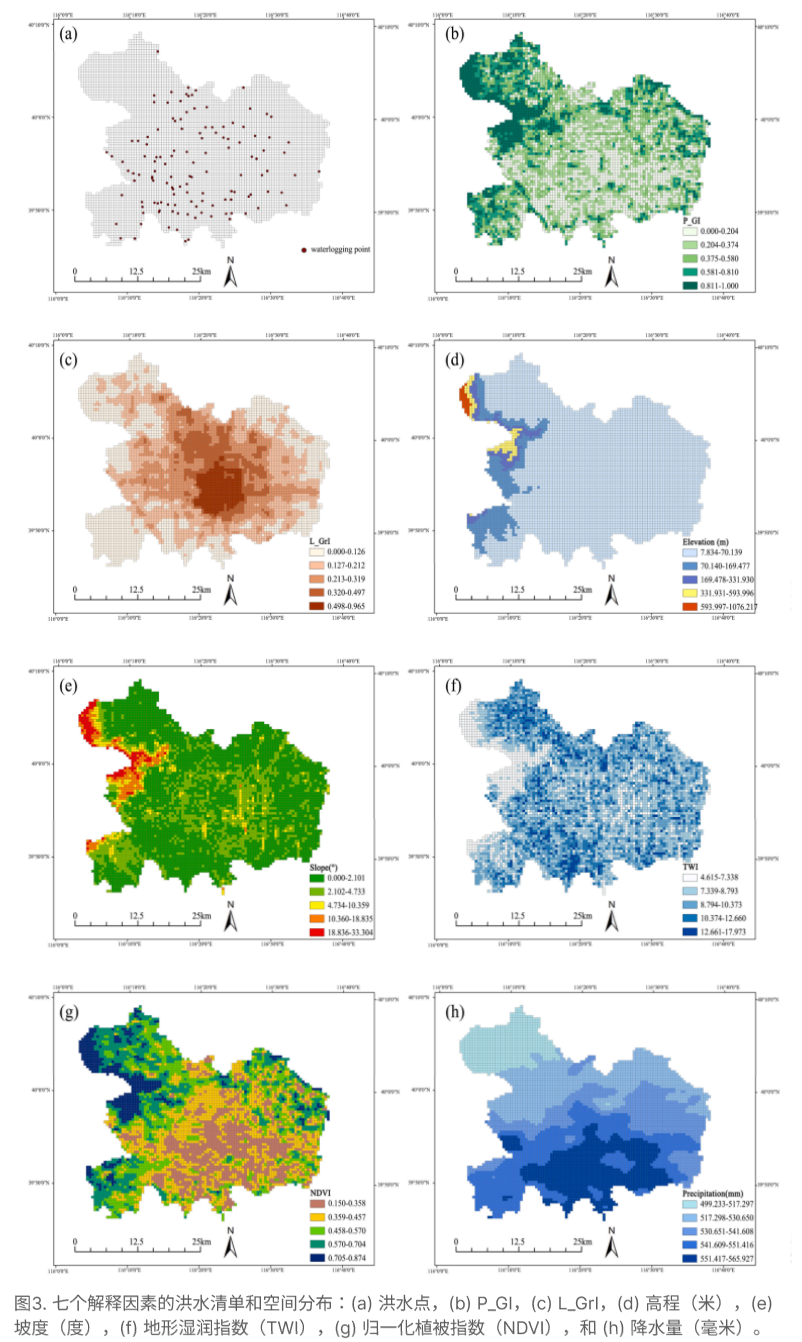
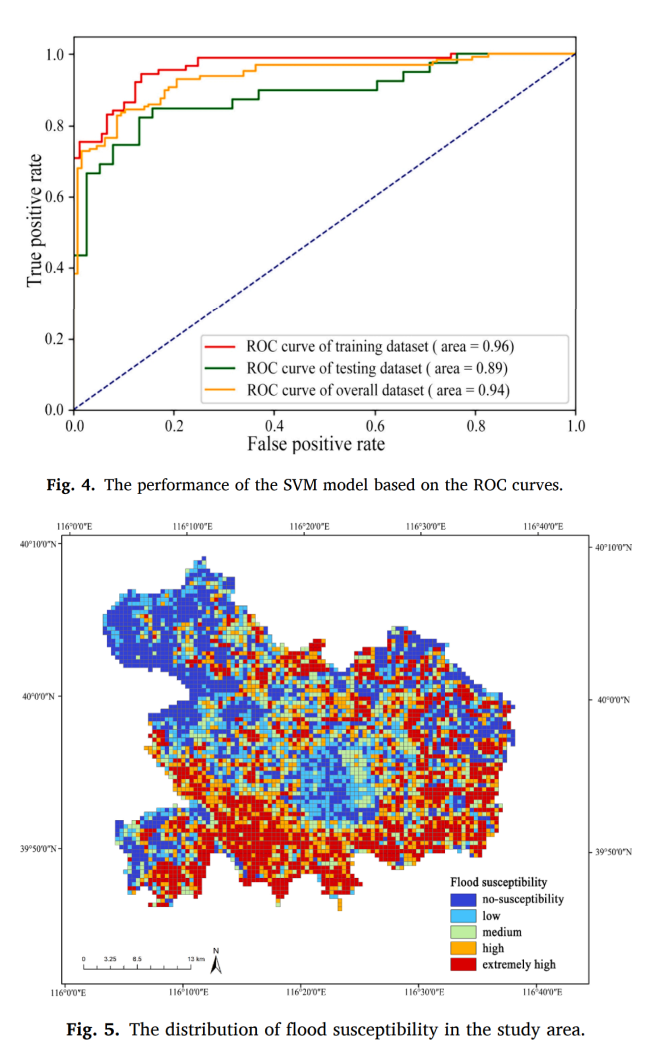
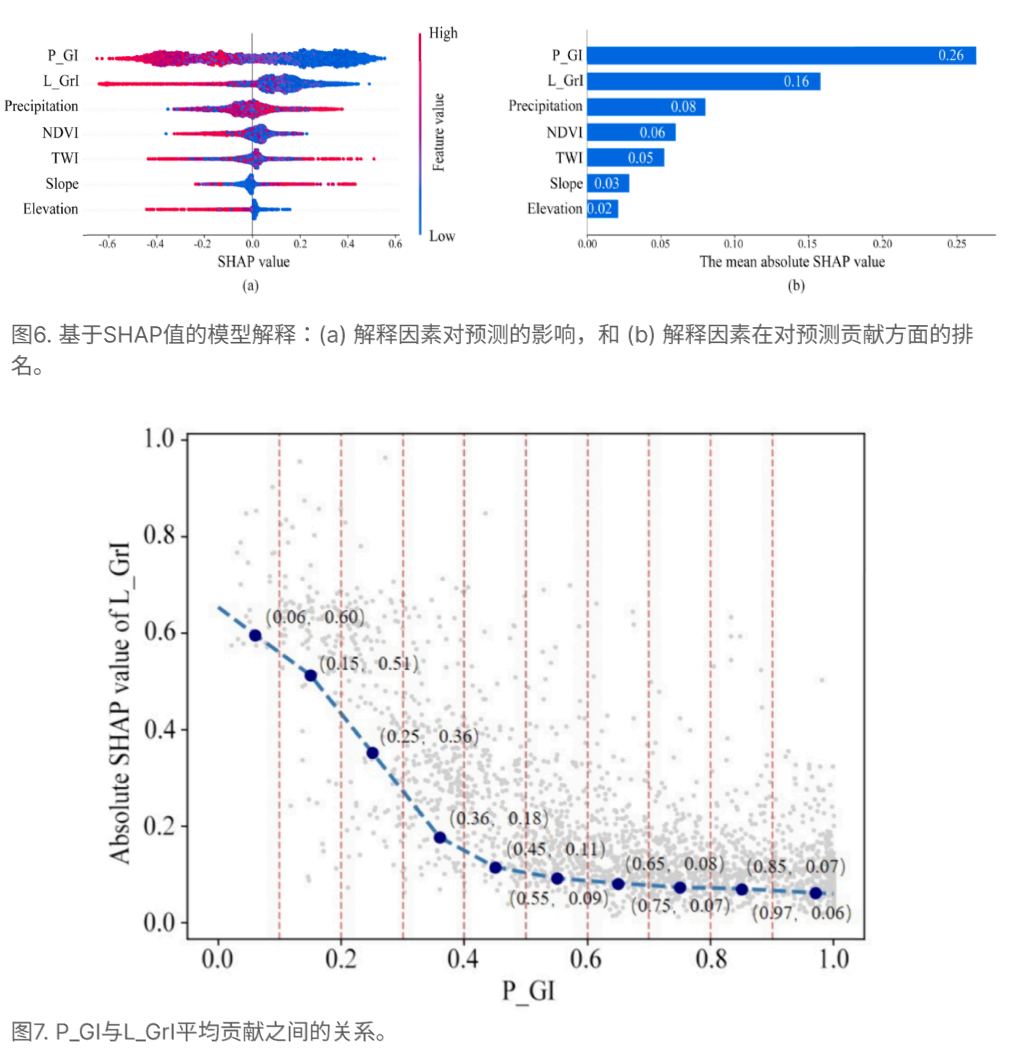
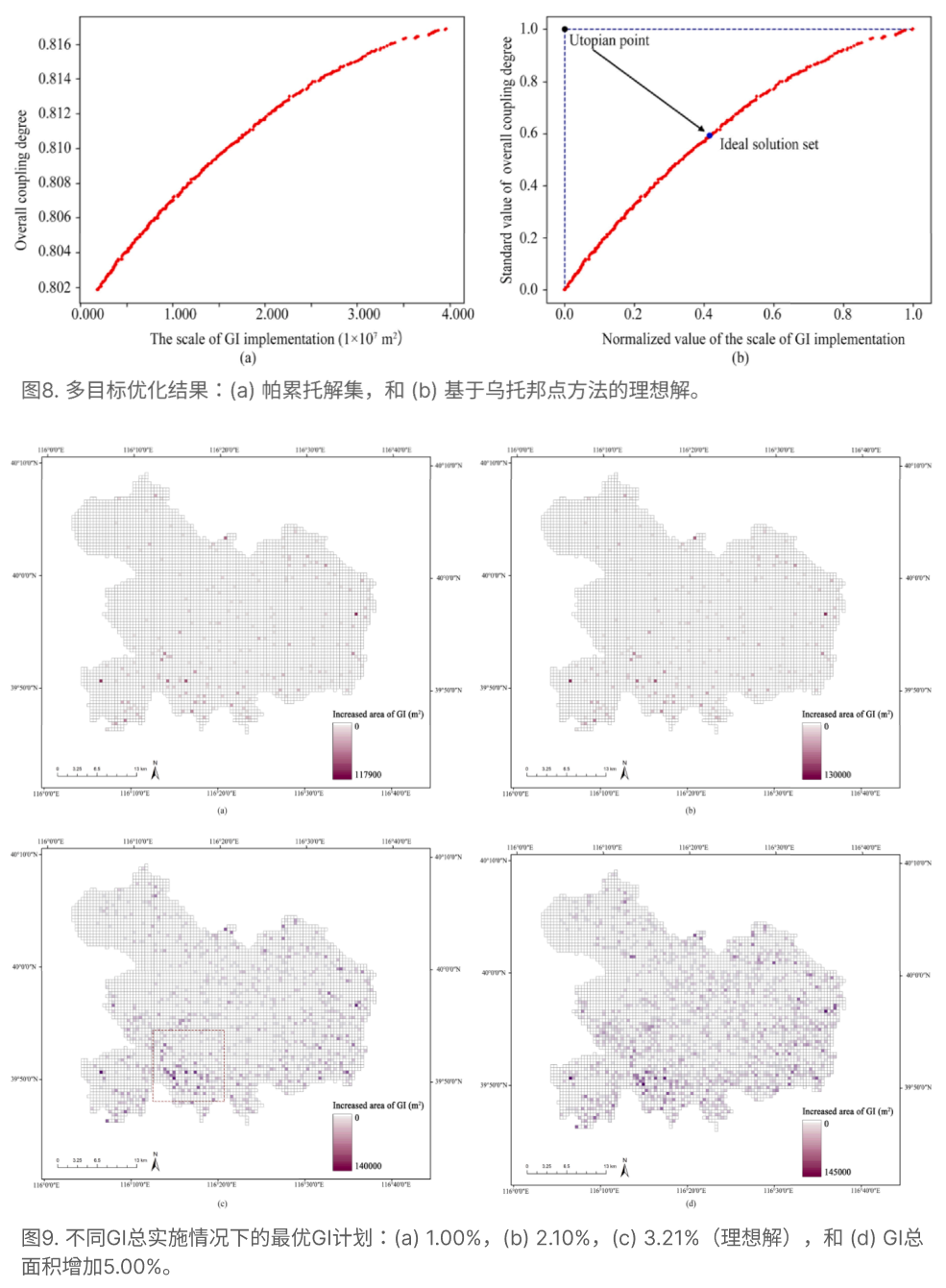