转自 数据派THU
本文约3800字,建议阅读7分钟
本文介绍了我们探讨Bayesian Bootstrap这个简单但功能强大的过程。
“自举”(翻译自bootstrap)这个词汇在多个领域可能见到,它字面意思是提着靴子上的带子把自己提起来,这当然是不可能的,在机器学习领域可以理解为原样本自身的数据再抽样得出新的样本及统计量,也有被翻译为自助法的。
Bayesian Bootstrap是一个强大的方法,它比其他的自举法更快,并且可以给出更紧密的置信区间,并避免许多极端情况。在本文中我们将详细地探讨这个简单但功能强大的过程。自举
自举是通过对数据进行随机重采样和替换来计算估计量属性的过程,它首先由Efron(1979)提出。这个过程非常简单,包括以下步骤:假设一个 i.i.d. 样本{Xᵢ}ᵢⁿ,并且我们想用估计量θ̂(X)计算一个统计θ。可以近似θ̂的分布如下:- 从样本{Xᵢ}ᵢⁿ中替换{X̃ᵢ}ᵢⁿ的n个观察样本。
这样θ̂-bootstrap的分布很好地逼近了θ̂的分布。首先,它很容易实现。因为我们只要重复做一件事情:估算θ,并且重复多次就可以了。这其实也是自举的一个主要缺点:如果评估过程很慢,那么自举法的计算成本就会变得很高。第二,自举不做分布假设。它只假设你的样本是总体的代表,观察结果是相互独立的。当观察结果彼此紧密联系时,比如在研究社交网络或市场互动时,可能会违反此假设。
当我们重新抽样时,我们所做的其实就是给我们的观察值分配整数权重,这样它们的和就等于样本容量n。这样的分布就是多项式分布。我们绘制大小为10.000的样本来看看多项式分布是什么样子的。从src.utils导入一些函数。 from src.utils import *
N = 10_000
np.random.seed(1)
bootstrap_weights = np.random.multinomial(N, np.ones(N)/N)
np.sum(bootstrap_weights)
#结果:10000
首先,我们确认权重之和是否确实等于1000,或者说,我们重采样生成了的是一个相同大小的数据。 sns.countplot(bootstrap_weights, color='C0').set(title='Bootstrap Weights');
正如我们所看到的,大约3600次观察得到的权重为零,而一些观察得到的权重为6。或者说大约3600个观察结果没有被重新采样,而一些观察结果被重采样多达6次。这里可能就有一个问题:为什么不用连续权值来代替离散权值呢?贝叶斯自举
Bayesian bootstrap是由Rubin(1981)提出的,它基于一个非常简单的想法:为什么不画一个更平滑的权重分布?多项式分布的连续等价是狄利克雷分布。下面的图绘制了一次观测的多项和狄利克雷权重的概率分布(它们分别是泊松分布和伽马分布)。第一个也是最直观的是,由于其连续的加权方案,它提供的估计值比普通的自举法更光滑。
此外连续加权方案阻止了极端情况的出现(没有观察到的0权重)。例如在线性回归中,如果原始样本中没有共线性,则不会出现共线性问题。最后作为一种贝叶斯方法:估计量的估计分布可以解释为具有非信息先验的后验分布。 bayesian_weights = np.random.dirichlet(alpha=np.ones(N), size=1)[0] * N
np.sum(bayesian_weights)
# 10000.000000000005
权重的总和(大约)为1,所以我们必须将它们乘以N。我们可以画出权重的分布,现在为了得到了连续的权重,我们必须近似分布。 sns.histplot(bayesian_weights, color='C1', kde=True).set(title='Dirichlet Weights');
狄利克雷分布有一个参数α,我们对所有观测值都设置为1。它是做什么的?α参数本质上决定被抽样的绝对概率和相对概率。增加所有观测值的α值可以减少分布的偏斜,使所有观测值具有更相似的权重。对于α→∞,所有的观测值得到相同的权重。那么我们应该如何选择α的值?Shao和Tu(1995)提出以下建议。The distribution of the random weight vector does not have to be restricted to the Diri(l, … , 1). Later investigations found that the weights having a scaled Diri(4, … ,4) distribution give better approximations (Tu and Zheng, 1987)
根据上面的建议,让我们来看看α=4的狄利克雷分布和之前α=1的狄利克雷分布是如何比较的。 bayesian_weights2 = np.random.dirichlet(np.ones(N) * 4, 1)[0] * N
sns.histplot(bayesian_weights, color='C1')
sns.histplot(bayesian_weights2, color='C2').set(title='Comparing Dirichlet Weights');
plt.legend([r'$\alpha = 1$', r'$\alpha = 4$']);
例子
首先,让我们看一看最简单、最常见的估计量:样本均值。首先我们从帕累托分布中得出100个观察值。 np.random.seed(2)
X = pd.Series(np.random.pareto(2, 100))
sns.histplot(X);
这种分布是非常倾斜的,几个观测值的值比平均值要高得多。 def classic_boot(df, estimator, seed=1):
df_boot = df.sample(n=len(df), replace=True, random_state=seed)
estimate = estimator(df_boot)
return estimate
def bayes_boot(df, estimator, seed=1):
np.random.seed(seed)
w = np.random.dirichlet(np.ones(len(df)), 1)[0]
result = estimator(df, weights=w)
return result
from joblib import Parallel, delayed
def bootstrap(boot_method, df, estimator, K):
r = Parallel(n_jobs=8)(delayed(boot_method)(df, estimator, seed=i) for i in range(K))
return r
def compare_boot(df, boot1, boot2, estimator, title, K=1000):
s1 = bootstrap(boot1, df, estimator, K)
s2 = bootstrap(boot2, df, estimator, K)
df = pd.DataFrame({'Estimate': s1 + s2,
'Estimator': ['Classic']*K + ['Bayes']*K})
sns.histplot(data=df, x='Estimate', hue='Estimator')
plt.legend([f'Bayes: {np.mean(s2):.2f} ({np.std(s2):.2f})',
f'Classic: {np.mean(s1):.2f} ({np.std(s1):.2f})'])
plt.title(f'Bootstrap Estimates of {title}')
compare_boot(X, classic_boot, bayes_boot, np.average, 'Sample Mean')
在这种情况下,这两个过程都给出了非常相似的结果。这两个分布非常接近,而且估计量的估计平均值和标准偏差几乎相同,与我们选择的自举无关。 import time
def compare_time(df, boot1, boot2, estimator, K=1000):
t1, t2 = np.zeros(K), np.zeros(K)
for k in range(K):
# Classic bootstrap
start = time.time()
boot1(df, estimator)
t1[k] = time.time() - start
# Bayesian bootstrap
start = time.time()
boot2(df, estimator)
t2[k] = time.time() - start
print(f"Bayes wins {np.mean(t1 > t2)*100}% of the time (by {np.mean((t1 - t2)/t1*100):.2f}%)")
Bayes wins 97.8% of the time (by 66.46%)
如果我们有一个不接受权重的估计量,例如中位数?我们可以进行两级抽样:我们采样权重,然后根据权重采样观测值。 def twolv_boot(df, estimator, seed=1):
np.random.seed(seed)
w = np.random.dirichlet(np.ones(len(df))*4, 1)[0]
df_boot = df.sample(n=len(df)*10, replace=True, weights=w, random_state=seed)
result = estimator(df_boot)
return result
np.random.seed(1)
X = pd.Series(np.random.normal(0, 10, 1000))
compare_boot(X, classic_boot, twolv_boot, np.median, "Sample Median")
可以看到,贝叶斯自举也比一般的自举更精确,因为α=4时的权重分布更密集。现在,看看一般的自举过程可能产生问题的案例。假设我们观察到一个正态分布的特征X和二元结果y。我们对两个变量之间的关系进行研究。 N = 100
np.random.seed(1)
x = np.random.normal(0, 1, N)
y = np.rint(np.random.normal(x, 1, N) > 2)
df = pd.DataFrame({'x': x, 'y': y})
df.head()
在该样本中,我们仅在100个观察结果中的到真值结果。由于结果是二进制的,因此可以使用逻辑回归模型。 smf.logit('y ~ x', data=df).fit(disp=False).summary().tables[1]
我们得到一个-23点的估计值,置信区间非常紧密的。
我们能自举估计量的分布吗?下面计算1000个自举样本的逻辑回归系数。
estimate_logit = lambda df: smf.logit('y ~ x', data=df).fit(disp=False).params[1]
for i in range(1000):
try:
classic_boot(df, estimate_logit, seed=i)
except Exception as e:
print(f'Error for bootstrap number {i}: {e}')
Error for bootstrap number 92: Perfect separation detected, results not available
Error for bootstrap number 521: Perfect separation detected, results not available
Error for bootstrap number 545: Perfect separation detected, results not available
Error for bootstrap number 721: Perfect separation detected, results not available
Error for bootstrap number 835: Perfect separation detected, results not available
对于1000个样本中的5个,我们无法计算估计值。但是这种情况是不会发生在贝叶斯自举过程中的。假设我们观察到二元特征X和连续的结果y。我们再次研究两个变量之间的关系。 N = 100
np.random.seed(1)
x = np.random.binomial(1, 5/N, N)
y = np.random.normal(1 + 2*x, 1, N)
df = pd.DataFrame({'x': x, 'y': y})
df.head()
estimate_beta = lambda df, **kwargs: smf.wls('y ~ x', data=df, **kwargs).fit().params[1]
compare_boot(df, classic_boot, bayes_boot, estimate_beta, "beta")
一般的自举过程估计的差异比贝叶斯自举大50%。为什么?如果我们更仔细地查看就会发现在将近20个重新采样的样本中,会得到一个非常不寻常的估计!这个问题的原因是在某些样本中,我们可能没有任何观察结果x = 1。因此在这些重采样的样本中,估计的系数为零。贝叶斯自举程序不会发生这种情况,因为它不会放弃任何观察(所有观察结果总是会包含所有的结果)。并且这里的一个重要的问题是我们没有收到任何错误消息或警告,这样我们很容易会忽视这个问题!总结
在本文中我们介绍了贝叶斯自举法, 它的关键的想法是,每当我们的估计量以加权估计量表示时,自举过程就等于用多项式权重随机加权。贝叶斯自举等同于用狄利克雷权重加权,这是多项式分布的连续等效物。具有连续的权重避免了极端的样本,并且可以生成估计量的平滑分布。本文参考
[1] B. Efron Bootstrap Methods: Another Look at the Jackknife (1979), The Annals of Statistics.
[2] D. Rubin, The Bayesian Bootstrap (1981), The Annals of Statistics.
[3] A. Lo, A Large Sample Study of the Bayesian Bootstrap (1987), The Annals of Statistics.
[4] J. Shao, D. Tu, Jacknife and Bootstrap (1995), Springer.
代码:
https://github.com/matteocourthoud/Blog-Posts/blob/main/notebooks/bayes_boot.ipynb
【免责声明】转载出于非商业性的教育和科研目的,只为学术新闻信息的传播,版权归原作者所有,如有侵权请立即与我们联系,我们将及时删除。
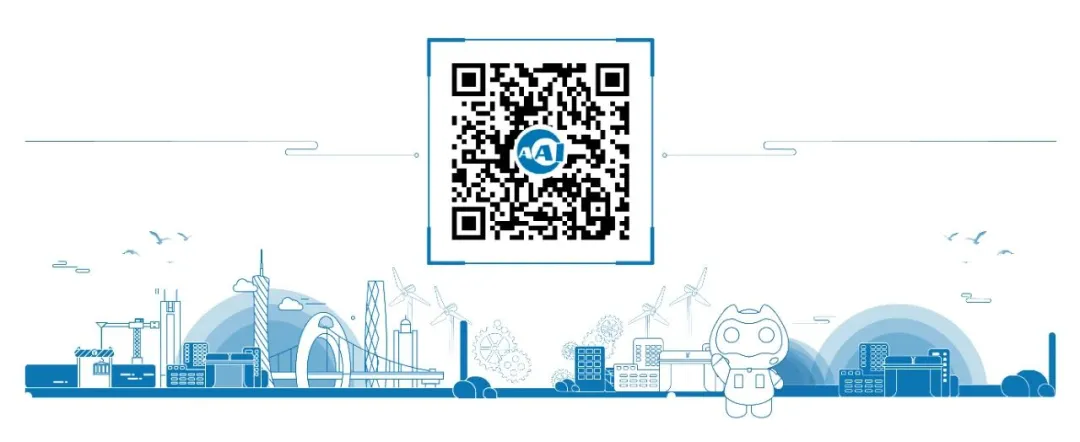