我们的新专辑《Github带有全套代码分享的文献复现2025》开启后受到大家的热烈喜爱,里面学习的文章为:《A multi-omic single-cell landscape of human gynecologic malignancies》,前面的学习笔记如下:
前面运行完后得到一个所有样本合并在一起的并去了批次的seurat对象:sce.all_int.qs,后面我又做了一个没有进行harmony的分析。
如果需要这个sce.all_int.qs
,可以加我的微信发给你:Biotree123
继续来学习wiki上的代码:https://github.com/RegnerM2015/scENDO_scOVAR_2020/wiki,今天进行细胞类型注释。
文献的注释结果
作者文献中的单细胞部分的细胞注释结果如下:总共有75523个细胞。
malignant clusters 鉴定策略:
- 使用的 the U.S. Food and Drug Administration (FDA)-approved biomarkers MUC16/CA125 and WFDC2/HE4 to identify EC and OC cancer clusters
- Expression of KIT/CD117 was used to identify GIST cancer clusters
- 结合inferCNV:nferred copy number variation (CNV) was used to help identify OC and GIST but not EC, as the disease rarely exhibits CNV
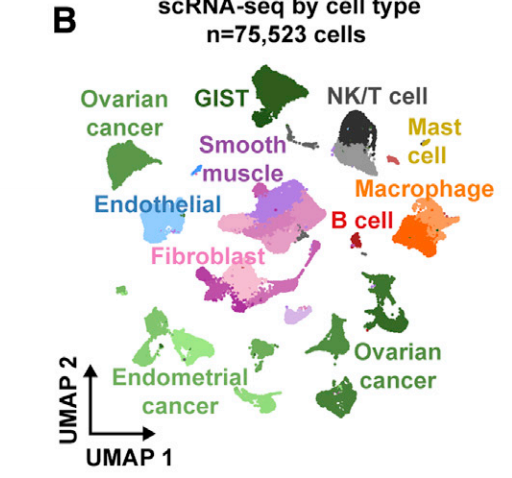
文章的附图:
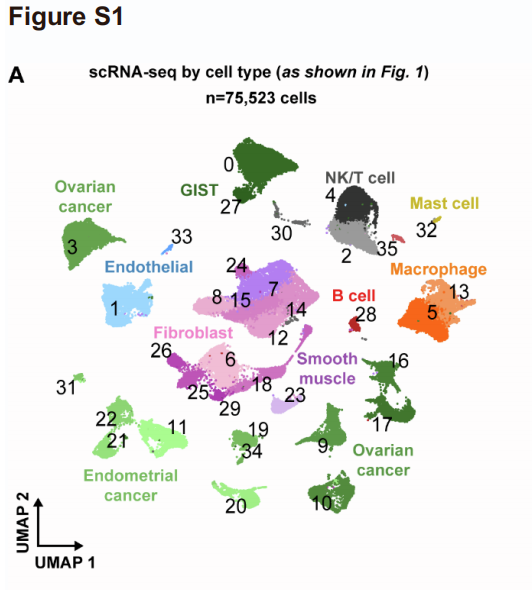
第一次尝试注释
作者的注释策略也比较复杂,我们先来看看使用常规的细胞已知marker尝试进行第一次注释。图中的细胞类型名称有:
- Endometrial cancer:MUC16/CA125
- NK/T cell:CD3D,CD3E,CD3G,CD2,KLRD1,KLRF1,NKG7
- B cell: CD19,MS4A1,CD79A,IGHG1
- Smooth muscle: ACTA2,MYH11
- Endothelial: RAMP2, PECAM1
- Fibroblast: DCN, LUM,COL1A2
先来看看没有进行harmony的注释结果。本次尝试使用res0.5的结果进行绘图,画一张markers气泡图:
rm(list=ls())
library(Seurat)
library(ggplot2)
library(SCP) # https://zhanghao-njmu.github.io/SCP/index.html
# https://scillus.netlify.app/vignettes/plotting
library(Scillus) # https://mp.weixin.qq.com/s/Z69GmXORqKczTsMQ68D4Vw
# https://samuel-marsh.github.io/scCustomize/
library(scCustomize)
library(qs)
###### step4: 看标记基因库 ######
# 原则上分辨率是需要自己肉眼判断,取决于个人经验
sce.all.int "2-harmony/sce.all_int-no.qs")
sce.all.int
table(Idents(sce.all.int))
table(sce.all.int$seurat_clusters)
table(sce.all.int$RNA_snn_res.0.1)
table(sce.all.int$RNA_snn_res.0.3)
table(sce.all.int$RNA_snn_res.0.5)
table(sce.all.int$RNA_snn_res.0.8)
getwd()
dir.create('3-check-by-0.5')
select_idet "RNA_snn_res.0.5"
sce.all.int$RNA_snn_res.0.5
#sce.all.int$RNA_snn_res.0.3
sce.all.int
table(sce.all.int@active.ident)
head(sce.all.int@meta.data)
# markers
cell_types
"Ovarian cancer" = c("WFDC2", "HE4"),
"GIST" = c("KIT", "CD117"),
"Endometrial cancer" = c("MUC16", "CA125"),
"NK/T cell" = c("CD3D", "CD3E", "CD3G", "CD2", "KLRD1", "KLRF1", "NKG7"),
"Mast cell" = c("TPSAB1", "TPSB2"),
"Macrophage" = c("CD163", "CD68"),
"B cell" = c("CD19", "MS4A1", "CD79A", "IGHG1"),
"Smooth muscle" = c("ACTA2", "MYH11"),
"Endothelial" = c("RAMP2", "PECAM1"),
"Fibroblast" = c("DCN", "LUM", "COL1A2"),
"Cell Cycle" = c("TOP2A", "MKI67"),
"Immu" = c("PTPRC")
)
p 'RNA',group.by = select_idet,cols = c("grey", "red") ) +
ggtitle(select_idet) +
xlab("") +
theme(axis.text.x = element_text(angle = 45, hjust = 1)) # 更改x轴标签角度
p[["theme"]][["strip.text"]]$angle
p
ggsave(filename = "3-check-by-0.5/Markers_dotplot-use.pdf", plot=p, width=13, height = 8,bg="white")
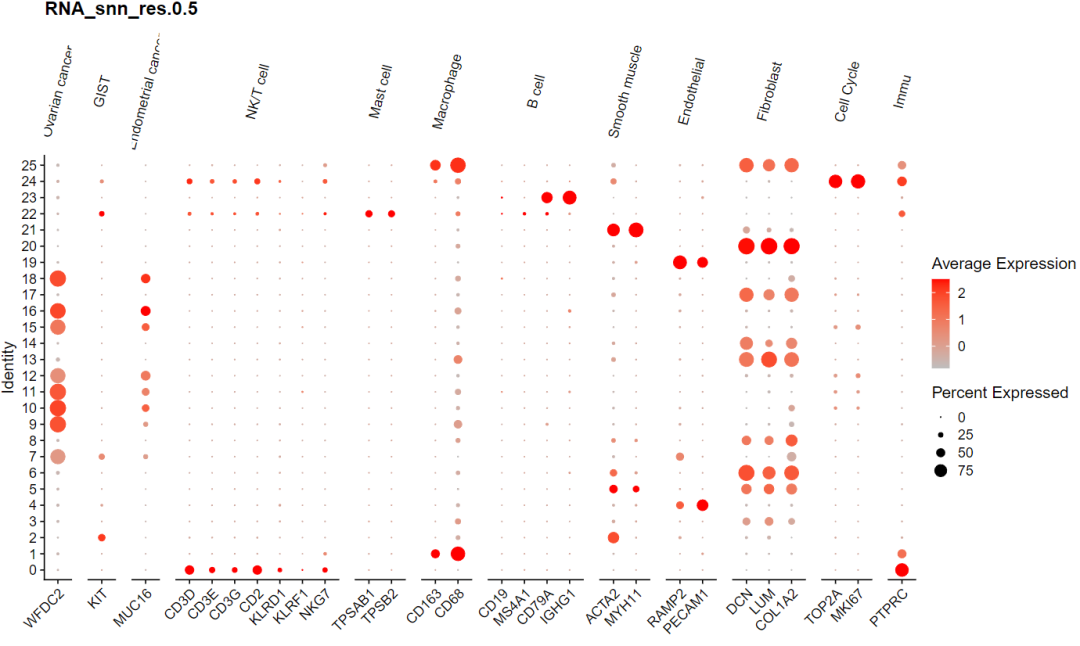
结合没有进行harmony的uamp图:
p "umap", group.by = select_idet, label = T) +
ggtitle(select_idet)
p
ggsave(plot=p, filename="3-check-by-0.5/Dimplot_resolution_0.5.pdf",width = 6, height = 6)
# 美化版
p "UMAP", label = T,label.size = 4, label_insitu = T,
label_point_size = 1, label_point_color =NA ,label_segment_color = NA)
p
ggsave(plot=p, filename="3-check-by-0.5/Dimplot_resolution_0.5-1.pdf",width = 7, height = 7)
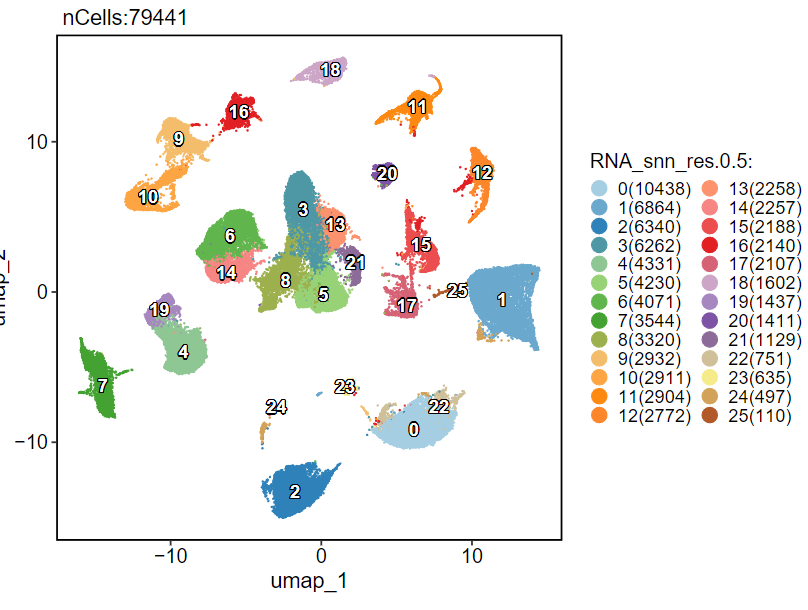
不同样本上色的umap:
p "umap",label=T,group.by = "orig.ident")
p
ggsave(filename='2-harmony/umap-by-orig.ident-before-harmony.png',plot = p, width = 6,height = 5.5)
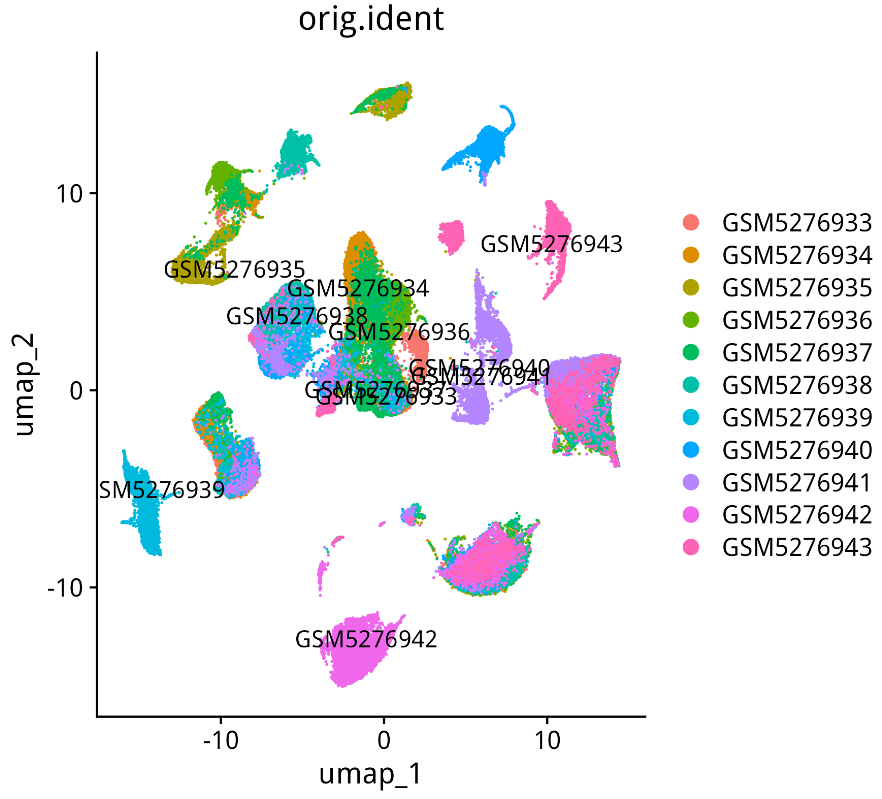
尝试第一次注释:
cluster2:https://www.ncbi.nlm.nih.gov/geo/query/acc.cgi?acc=GSM5276942,为单独一个样本,GIST类型,表达 KIT,注释为 GIST
cluster7:https://www.ncbi.nlm.nih.gov/geo/query/acc.cgi?acc=GSM5276939,为单独一个样本,Endometrioid类型,表达上皮marker,以及WFDC2,注释为 Ovarian cancer
其余问号这里现在还没有确定,我需要再找一些信息辅助进来,cluster25感觉更像是双细胞,还有mast那一群很奇怪,它跟NK/T挤在了一起没有分开,而Fibroblast 则是分散成了很多群。
0 | NK/T cell | 10 | Ovarian cancer | 20 | Fibroblast |
1 |
| | | | |
2 | | | | | |
3 |
| | | | |
4 | | | | | |
5 | | | | | |
6 | | | | | |
7 | | | | | |
8 | | | | | |
9 | | | | | |
(未完待续~)
文末友情宣传