推荐普林斯顿大学土木与环境工程系主任Catherine Peters教授团队的工作。 “编辑精选 Editor's Choice” 论文获得开放获取(Open Access)半年的福利。点击文末“阅读原文”,即可直达。
本文是数字岩心技术的一项突破性进展,被缩写为SMART Mapping (Synchrotron-based Machine learning Approach for RasTer),即用基于同步辐射光谱技术(荧光或衍射)数据集训练神经网络,并可用于大尺度、快速的矿物识别和时空重构。本文用详细的数据集案例证明了基于同步辐射的二维矿物扫描图可以提供矿物丰度、痕量元素、粒度和孔隙可及性等信息。论文写作流畅,功底深厚,得到了审稿人和编辑的一致好评。
本文发表在AG期刊与国际多孔介质学会(INTERPORE)联合主办的Applied Geochemistry in Porous Media的专刊(Virtual Special Issue)中。该专刊质量上乘,充分体现了期刊+学会+会议三者之间的联动。类似的联动还有在火热征稿的AG/IAP(Interfaces Against Pollution)专刊。
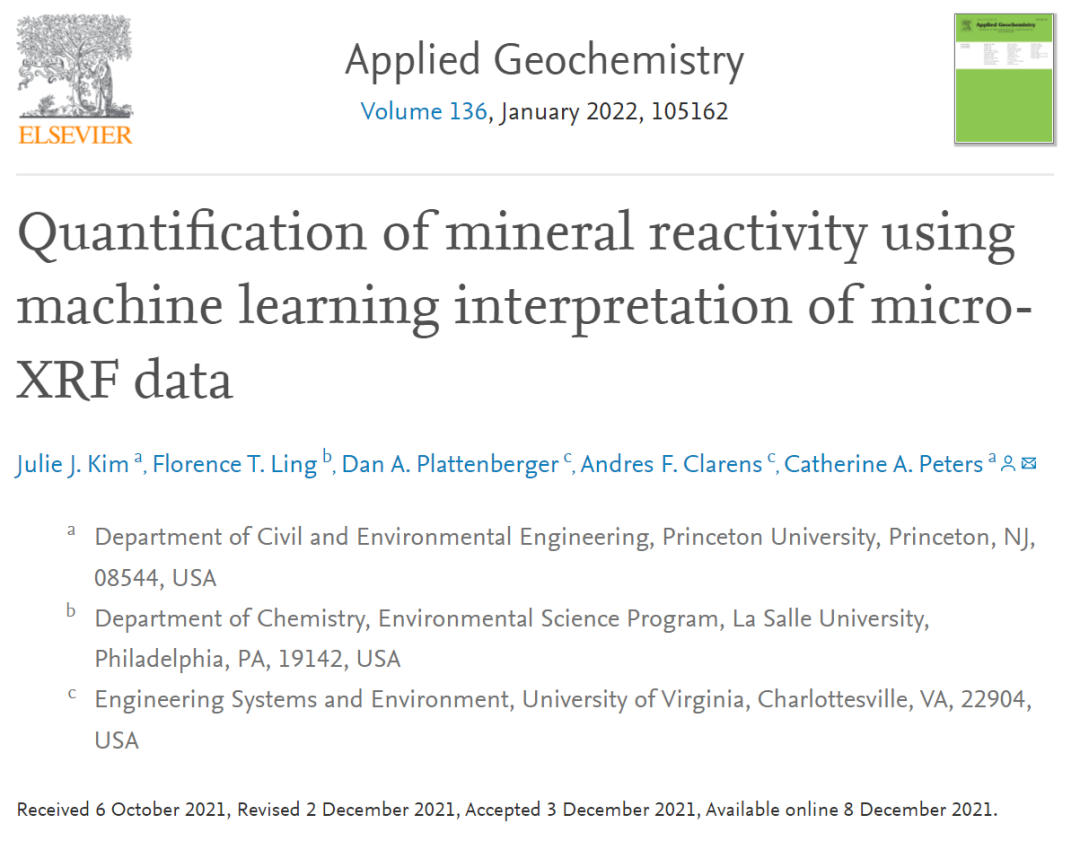
Highlights
A trained SMART classifier is extended by pooling synchrotron XRF datasets.
7-mineral classifier identifies and maps minerals with <4% misclassification rate.
mm-scale vein and consolidated shale rock samples are mineralogically mapped.
Pyrite in Eagle Ford shale is characterized for its grain size, and trace elements.
Mineral accessibility is quantified by adjacency to reactive mineral phases.
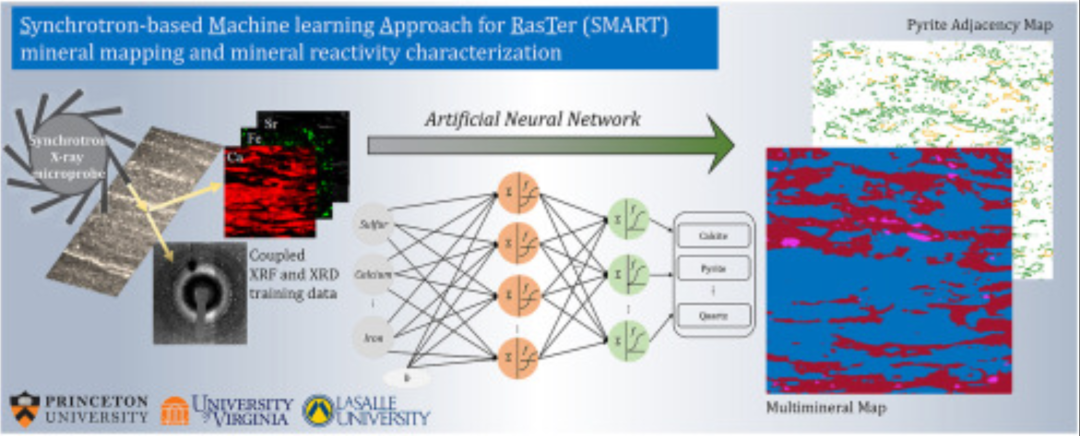
Abstract
Accurate characterizations of mineral reactivity require mapping of spatial heterogeneity, and quantifications of mineral abundances, elemental content, and mineral accessibility. Reactive transport models require such information at the grain-scale to accurately simulate coupled processes of mineral reactions, aqueous solution speciation, and mass transport. In this work, millimeter-scale mineral maps are generated using a neural network approach for 2D mineral mapping based on synchrotron
micro x-ray fluorescence (μXRF) data. The approach is called Synchrotron-based Machine learning Approach for RasTer (SMART) mapping, which reads μXRF scans and provides mineral maps of the same size and resolution. The SMART mineral classifier is trained on coupled μXRF and micro-x-ray diffraction (μXRD) data, which is what distinguishes it from existing mapping tools. Here, the SMART classifier was applied to μXRF scans of various sedimentary rock samples including consolidated shales from the Eagle Ford (EFS1), Green River (GRS1), Haynesville (HS1), and New Albany (NAS1) formations and a syntaxial vein from the Upper Wolfcamp formation. The data were obtained using an x-ray microprobe at beamline 13-ID-E at the Advanced Photon Sources. Individual mineral maps generated by the SMART classifier well-captured distributions of both dominant and minor phases in the shale rocks and revealed EFS1 and GRS1 to be carbonate rich shales, and NAS1 and HS1 to be sulfide rich shales. The EFS1 was further characterized for its trace mineral abundances, grain sizes, trace element composition, and accessibility. Approximately 4.4 wt% of the rock matrix were found to be pyrite, with a median grain size of 3.17 μm in diameter and 62% of the grains predicted to be smaller than 4 μm. Quantifications of trace elements in pyrite revealed zinc concentrations up to 4.2 wt%, along with minor copper and arsenic copresence. Mineral accessibility was examined by contact with other phases and was quantified using a new type of image we are calling an adjacency map. Adjacency analyses revealed that of the total pyrite surface present in the EFS1, 28% is in contact with calcite. The adjacency maps are useful for quantifying the likelihood that a mineral could be exposed to fluids after dissolution of a contacting reactive phase like calcite. Lastly, pooling data from different samples was demonstrated by training a classifier using two sets of coupled μXRF-μXRD data. This classifier yielded an overall accuracy of >96%, demonstrating that data pooling is a promising approach for applications to a wide suite of rock samples of different origin, size, and thickness.
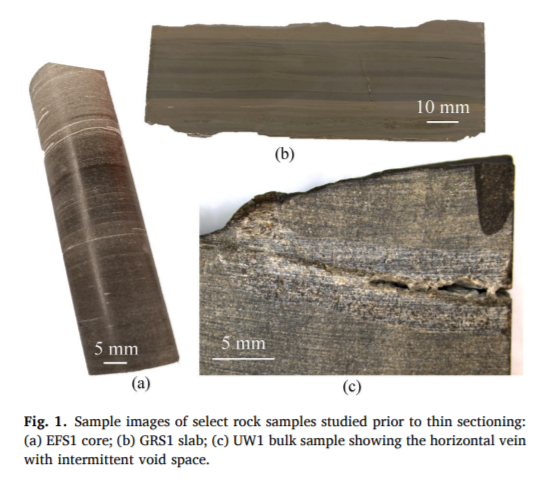
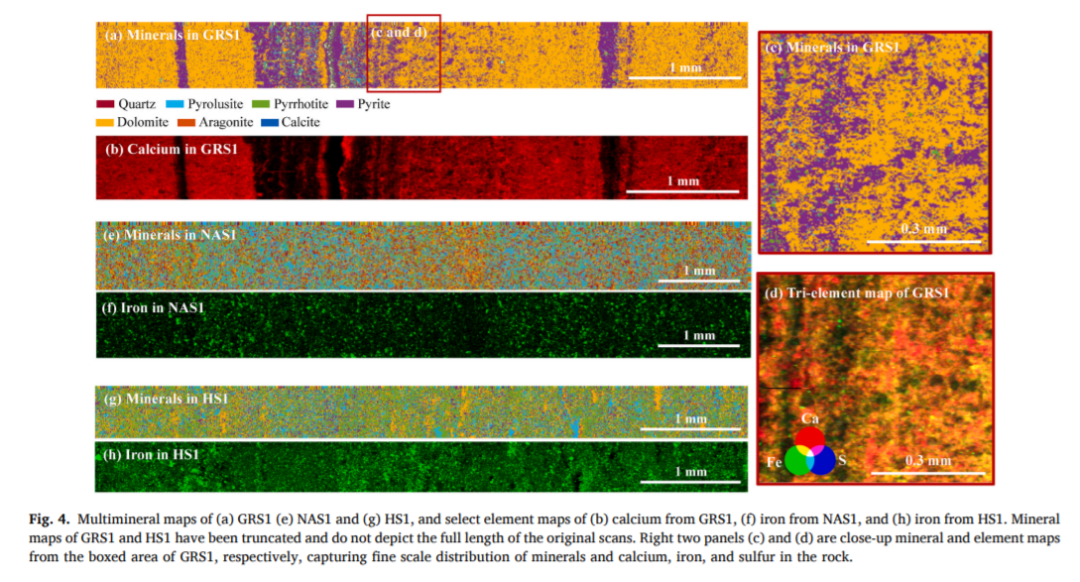
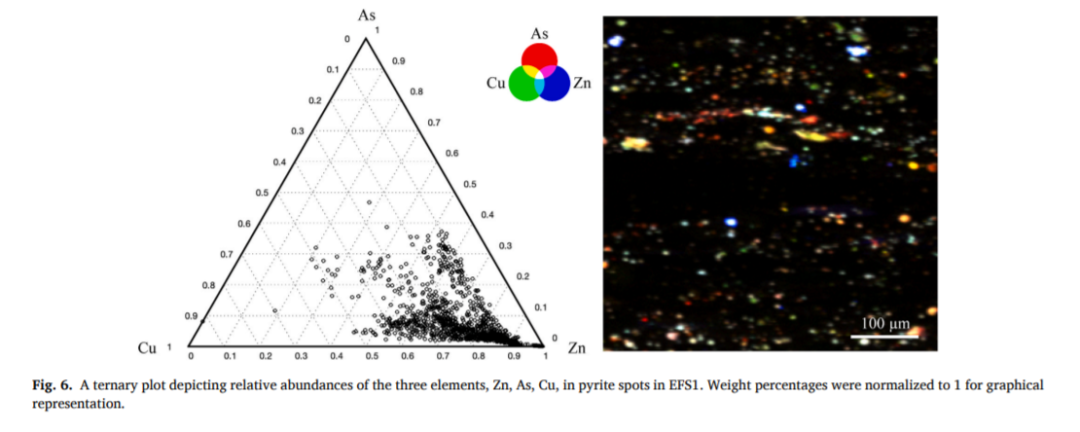
来源:AG期刊。投稿、合作、转载、进群,请添加小编微信Environmentor2020!环境人Environmentor是环境领域最大的学术公号,拥有近10W活跃读者。由于微信修改了推送规则,请大家将环境人Environmentor加为星标,或每次看完后点击页面下端的“在看”,这样可以第一时间收到我们每日的推文!环境人Environmentor现有综合群、期刊投稿群、基金申请群、留学申请群、各研究领域群等共20余个,欢迎大家加小编微信Environmentor2020,我们会尽快拉您进入对应的群。