网页地址:http://t.cn/A67rUJxO
数据包含近229万条记录和109个字段,以下取较重要的字段进行说明。
user_id:用户编码,用户唯一标识
bd_stronghold_level:要塞等级,相当于游戏账号等级
wood_reduce_value:木头消耗数量
stone_reduce_value:石头消耗数量
ivory_reduce_value:象牙消耗数量
meat_reduce_value:肉消耗数量
magic_reduce_value:魔法消耗数量
general_acceleration_reduce_value:通用加速消耗数量
building_acceleration_reduce_value:建筑加速消耗数量
reaserch_acceleration_reduce_value:科研加速消耗数量
training_acceleration_reduce_value:训练加速消耗数量
treatment_acceleration_reduce_value:治疗加速消耗数量
pvp_battle_count:玩家对玩家次数
pve_battle_count:玩家对机器次数
avg_online_minutes:日均在线时间
pay_price : 消费金额
pay_count:消费次数
用户注册时间分布情况?
用户的付费情况(付费率,ARPU,ARPPU)?
各等级用户的付费情况?
用户的消费习惯?
1. 导入数据
import numpy as np
import pandas as pd
from pandas import read_csv
from sklearn.cluster import KMeans
import matplotlib.pyplotas plt
import pylab as pl
from matplotlib.font_managerimport FontManager, FontProperties
pd.set_option('display.max_columns',None)
df=df0
print(df.isnull().any().any())
print(df.head())
2. 清洗数据
df=df.drop_duplicates(subset='user_id')
print('用户总数:',len(df['user_id']))
→用户总数:2288007
3. 计算用户注册时间分布
register_date=[]
for i in df['register_time']:
date=i[5:10]
register_date.append(date)
df['register_time']=register_date
df_register=df.groupby('register_time').size()
df_register.columns=['日期','注册人数']
print(df_register)
(可视化)
plt.plot(df_register)
plt.grid(True)
pl.xticks(rotation=90)
font=FontProperties(fname='/System/Library/Fonts/PingFang.ttc')
plt.title('用户注册分布图',fontproperties=font)
plt.show()
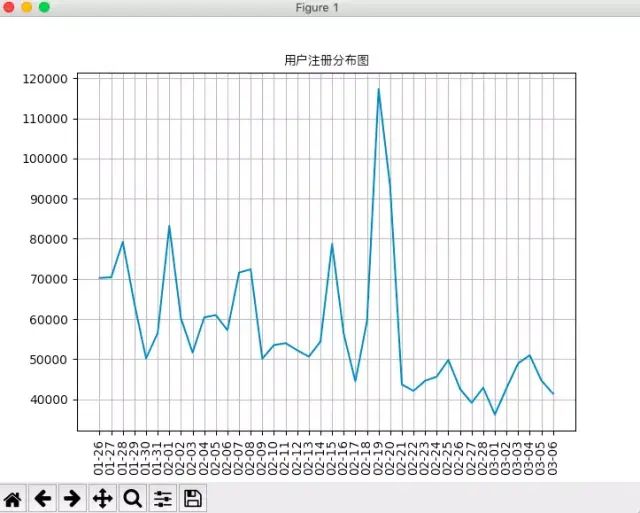
由上图可以看出,用户注册数在2月19日有一次的大的高峰,其他时间也分别有几次小高峰,且高峰的持续时间很短,可以推测是因为游戏推出一些奖励活动或公司对游戏的推广取得了效果进而使注册用户激增。
4. 用户的付费情况(付费率,ARPU,ARPPU)
df_pay_user=df[(df['pay_price']>0)]
pay_rate=df_pay_user['user_id'].count()/df_active_user['user_id'].count()
print('付费率:%.2f'%(pay_rate))
arpu=df_pay_user['pay_price'].sum()/df_active_user['user_id'].count()
print('ARPU:%.2f'%(arpu))
arppu=df_pay_user['pay_price'].sum()/df_pay_user['user_id'].count()
print('ARPPU:%.2f'%(arppu))
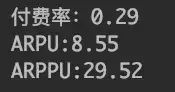
由上图可知目前盈利较好的手游的ARPU超过5元,一般手游在3~5元之间,盈利较差的低于3元,该游戏的ARPU为8.55元,说明盈利水平较高。
5. 不同等级用户的付费情况
df_user=df[['user_id','bd_stronghold_level','pay_price','pay_count']]
df_table=pd.pivot_table(df_user,index=['bd_stronghold_level'],
values=['user_id','pay_price','pay_count'],
aggfunc={'user_id':'count','pay_price':'sum','pay_count':'sum'})
df_stronghold_pay=pd.DataFrame(df_table.to_records())
df_stronghold_pay['pay_num']=df_user[(df_user['pay_price']>0)].groupby('bd_stronghold_level').user_id.count()
df_stronghold_pay['pay_rate']=df_stronghold_pay['pay_num']/df_stronghold_pay['user_id']
df_stronghold_pay['avg_pay_price']=df_stronghold_pay['pay_price']/df_stronghold_pay['user_id']
df_stronghold_pay['avg_pay_count']=df_stronghold_pay['pay_count']/df_stronghold_pay['user_id']
df_stronghold_pay.columns=['要塞等级','总付费次数','总付费金额','总人数',
'付费人数','付费转化率','人均付费金额','人均付费次数']
df_stronghold_pay=df_stronghold_pay[['要塞等级','总人数','付费人数','付费转化率',
'总付费金额','人均付费金额','总付费次数','人均付费次数']]
df_stronghold_pay=df_stronghold_pay.round(2)
print(df_stronghold_pay)
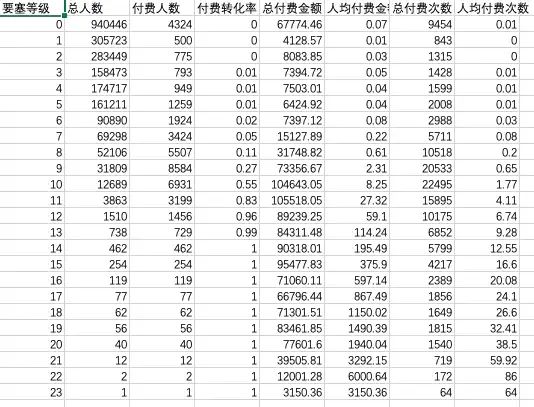
可视化:
x=df_stronghold_pay['要塞等级']
y=df_stronghold_pay['付费转化率']
plt.xticks(x,range(0,len(x),1))
plt.plot(x,y)
plt.grid(True)
plt.title('不同等级用户付费转化率',fontproperties=font)
plt.show()
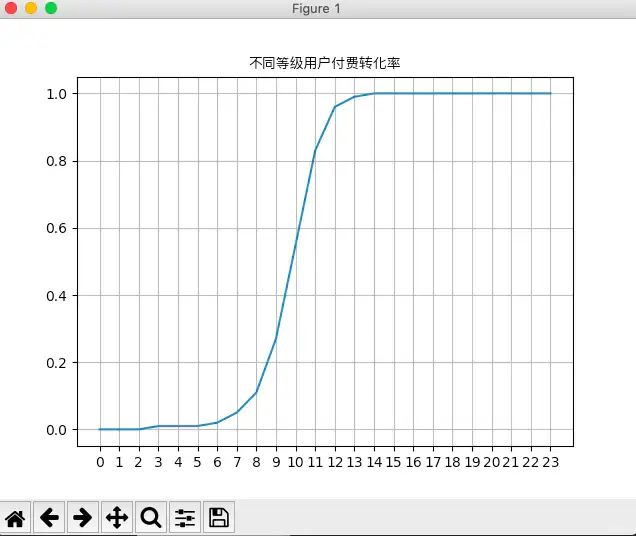
x=df_stronghold_pay['要塞等级']
y=df_stronghold_pay['人均付费金额']
plt.xticks(x,range(0,len(x),1))
plt.plot(x,y)
plt.grid(True)
plt.title('不同等级用户人均付费jine',fontproperties=font)
plt.show()
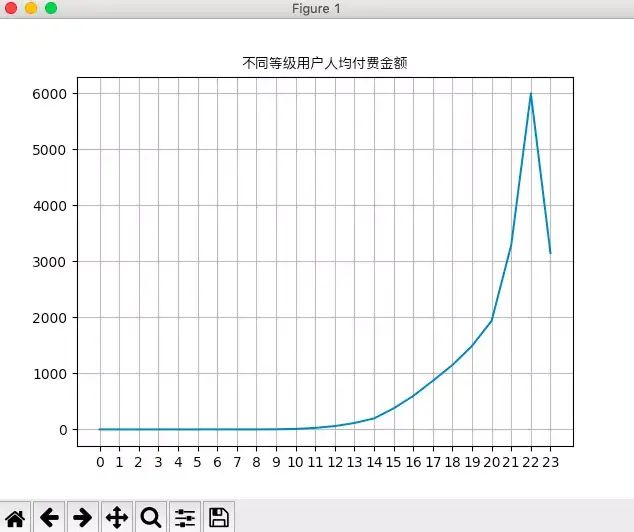
x=df_stronghold_pay['要塞等级']
y=df_stronghold_pay['人均付费金额']
plt.xticks(x,range(0,len(x),1))
plt.plot(x,y)
plt.grid(True)
plt.title('不同等级用户人均付费jine',fontproperties=font)
plt.show()
#要塞等级-人均付费次数
x=df_stronghold_pay['要塞等级']
y=df_stronghold_pay['人均付费次数']
plt.xticks(x,range(0,len(x),1))
plt.plot(x,y)
plt.grid(True)
plt.title('不同等级用户人均付费次数',fontproperties=font)
plt.show()
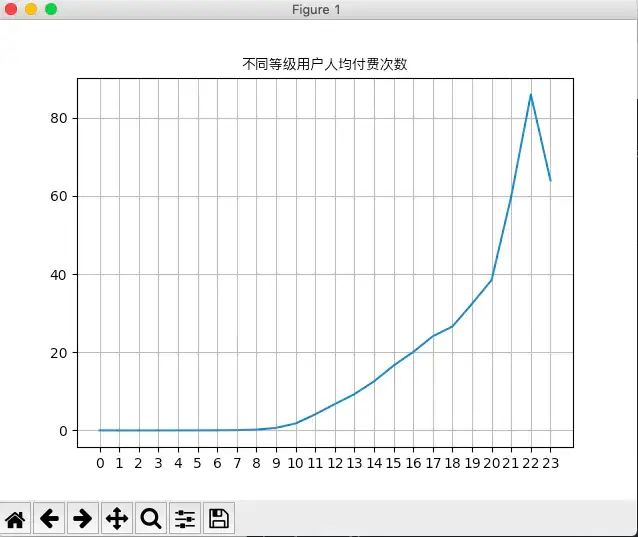
从上面看到用户等级到达10级时,付费率接近60%,等级到达13级时,付费率接近100%,且人均付费金额和次数两项指标也在用户达到10级后增长迅速,因此可以认定10级以上用户为游戏的核心用户。
但是观察用户等级分布,发现绝大部分用户还是处在10级以下的水平,因此如何使用户达到10级是游戏运营接下来需要考虑的事。
6. 不同玩家的消费习惯
该游戏充值主要可以获得道具类(木头、石头、象牙、肉、魔法)和加速券类(通用、建筑、科研、训练、医疗)。根据用户的充值金额大小,分别分析两类消费品的消耗情况。
df_eli_user=df[(df['pay_price']>=500)&(df['bd_stronghold_level']>=10)]
df_nor_user=df[(df['pay_price']<500)&(df['bd_stronghold_level']>10)]
wood_avg=[df_eli_user['wood_reduce_value'].mean(),df_nor_user['wood_reduce_value'].mean()]
stone_avg=[df_eli_user['stone_reduce_value'].mean(),df_nor_user['stone_reduce_value'].mean()]
ivory_avg=[df_eli_user['ivory_reduce_value'].mean(),df_nor_user['ivory_reduce_value'].mean()]
meat_avg=[df_eli_user['meat_reduce_value'].mean(),df_nor_user['meat_reduce_value'].mean()]
magic_avg=[df_eli_user['magic_reduce_value'].mean(),df_nor_user['magic_reduce_value'].mean()]
props_data={'high_value_player':[wood_avg[0],stone_avg[0],ivory_avg[0],meat_avg[0],magic_avg[0]],
'normal_player':[wood_avg[1],stone_avg[1],ivory_avg[1],meat_avg[1],magic_avg[1]]}
df_props=pd.DataFrame(props_data,index=['wood','stone','ivory','meat','magic'])
df_props=df_props.round(2)
print(df_props)
ax=df_props.plot(kind='bar',title='Props Reduce',
grid=True,legend=True)
plt.show()
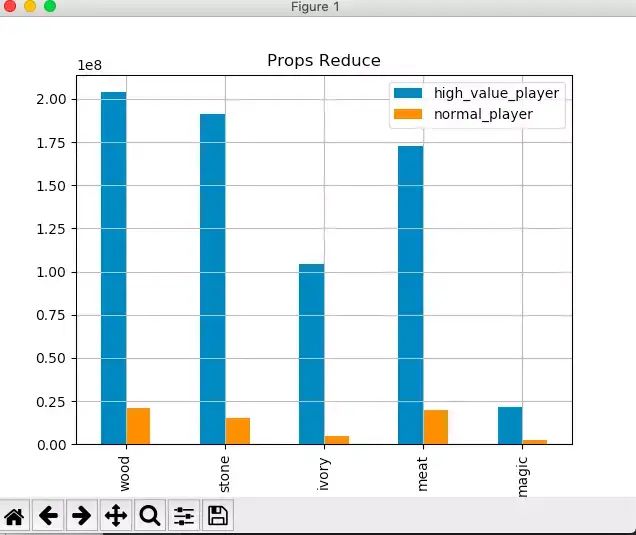
普通玩家和高消费玩家对木头、石头、肉的消耗都较大,魔法的消耗都较小,而在象牙的消耗上,高消费玩家和普通玩家的消耗差距较大。
general_avg=[df_eli_user['general_acceleration_reduce_value'].mean(),
df_nor_user['general_acceleration_reduce_value'].mean()]
building_avg=[df_eli_user['building_acceleration_reduce_value'].mean(),
df_nor_user['building_acceleration_reduce_value'].mean()]
research_avg=[df_eli_user['reaserch_acceleration_reduce_value'].mean(),
df_nor_user['reaserch_acceleration_reduce_value'].mean()]
training_avg=[df_eli_user['training_acceleration_reduce_value'].mean(),
df_nor_user['training_acceleration_reduce_value'].mean()]
treatment_avg=[df_eli_user['treatment_acceleration_reduce_value'].mean(),
df_nor_user['treatment_acceleration_reduce_value'].mean()]
acceleration_data={'high_value_player':[general_avg[0],building_avg[0],research_avg[0],
training_avg[0],treatment_avg[0]],
'normal_player':[general_avg[1],building_avg[1],research_avg[1],
training_avg[1],treatment_avg[1]]}
df_acceleration=pd.DataFrame(acceleration_data,index=['general','building','researching','training','treatment'])
print(df_acceleration.round(2))
ax=df_acceleration.plot(kind='bar',title='Acceleration Reduce',
grid=True,legend=True)
plt.show()
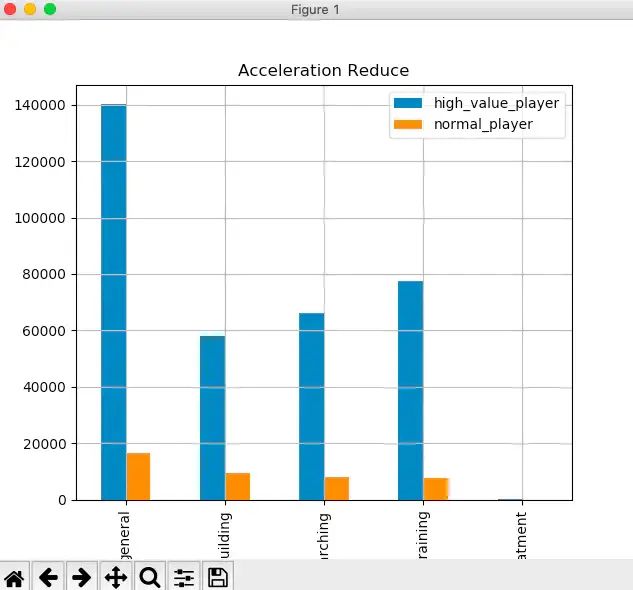
从上图看出两类玩家对对治疗加速券消耗都很小,对通用加速券的消耗差异较大,其他三种加速券消耗差别不大。
1. 该游戏具有较大的用户基数,且新用户注册受游戏活动、新版本等因素影响较大。
2. 该游戏的ARPU为8.55,说明该游戏的盈利能力较高。
3. 用户等级达到10级后,付费意愿明显上升,且达到13级时付费率将近100%。但是绝大多数用户仍然停留在10级以下,如何使用户平滑升至10级尤为重要。
4. 消费习惯上,高消费玩家对象牙和通用加速券的需求远多于一般玩家。