数据集制作
我用手机拍了一张图像
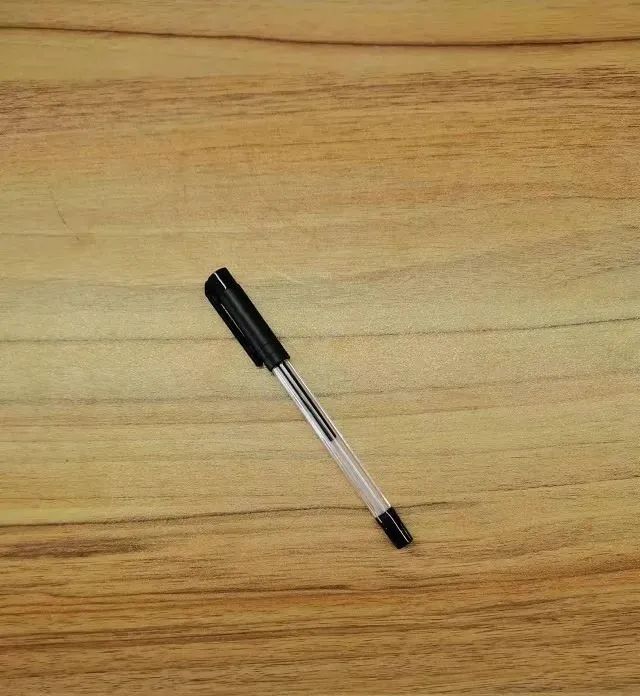
然后自己写个代码,每旋转一度保存一张图像,这样就成功生成了360张图像及其注释文件,分为训练集与验证集。训练文件夹包含 320张带有注释的图像。测试和验证文件夹都包含 40 张带有注释的图像。数据集部分图像显示如下:
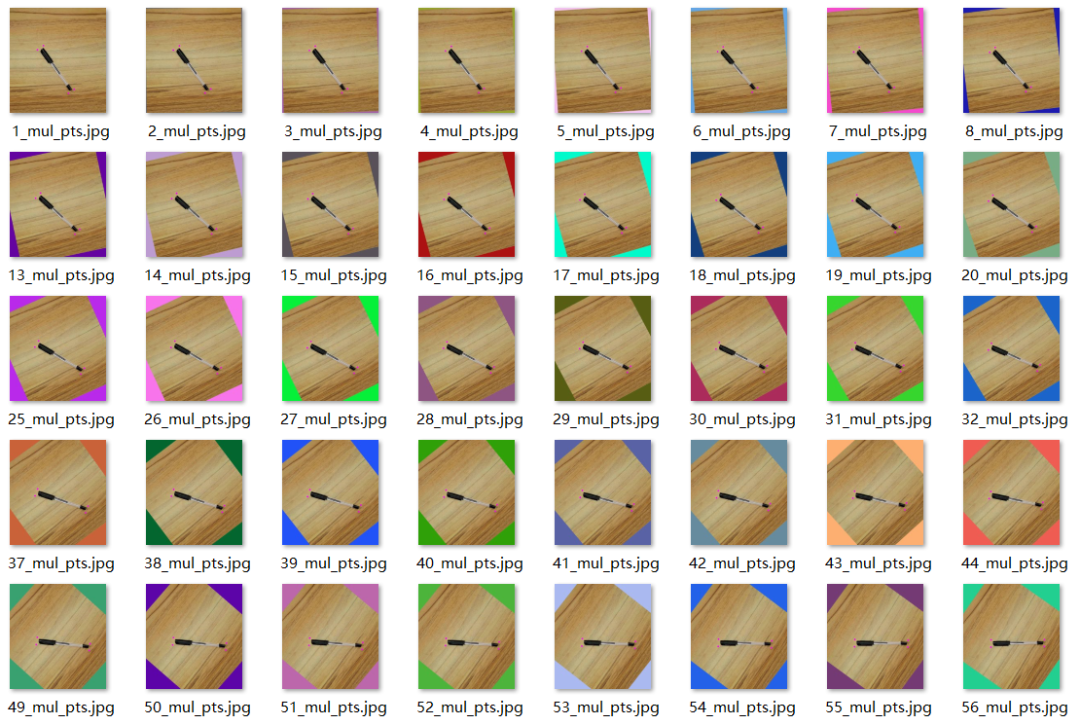
模型训练
准备好数据集以后,直接按下面的命令行运行即可:
yolo obb train data=pen_dataset.yaml model=yolov8s-obb.pt epochs=25 imgsz=640
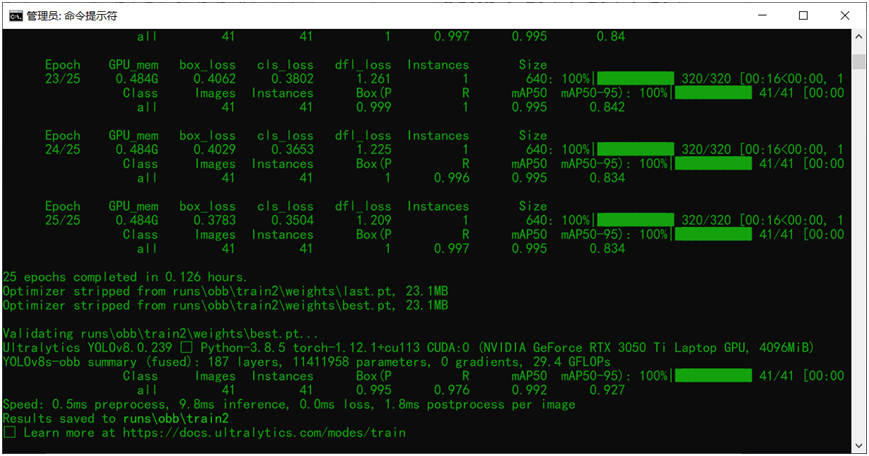
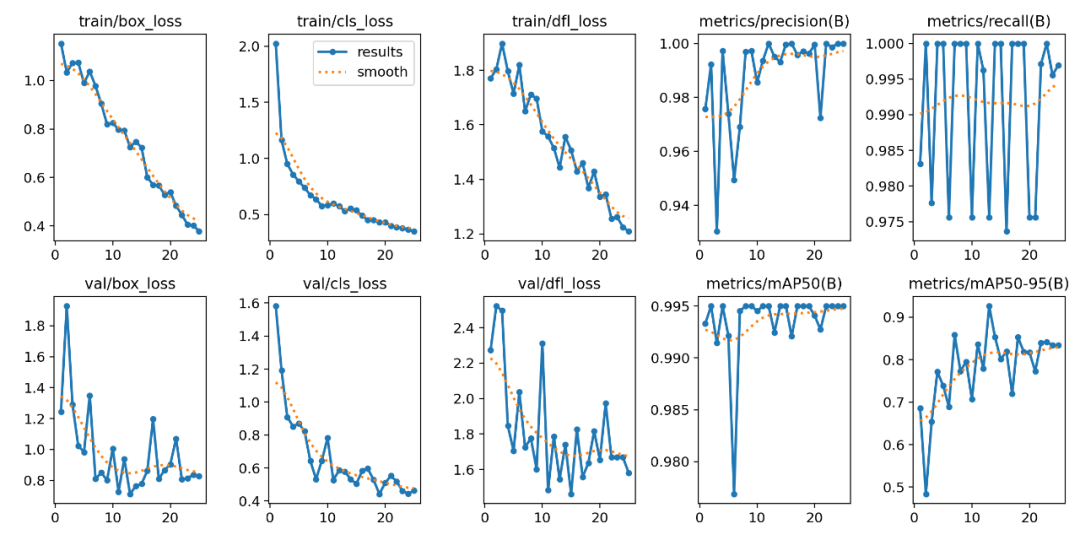
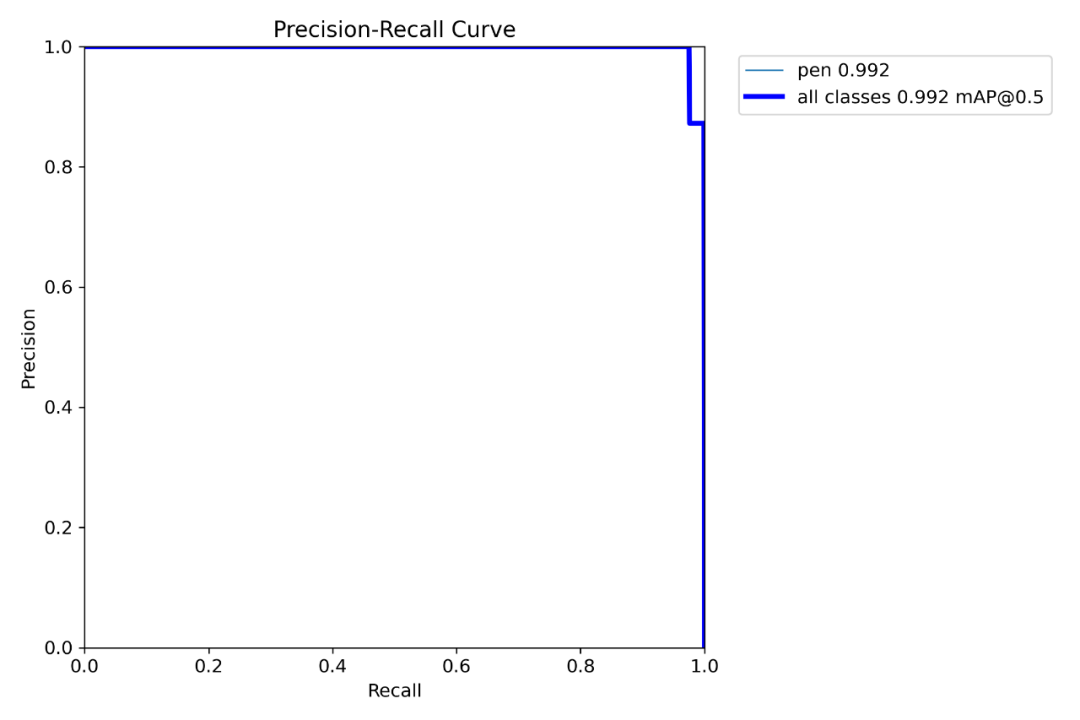
导出与测试
模型导出与测试
# export model
yolo export model=yolov8s-obb.pt format=onnx
# inference model
yolo obb predict model=pen_best.pt source=pen_rotate_test.png
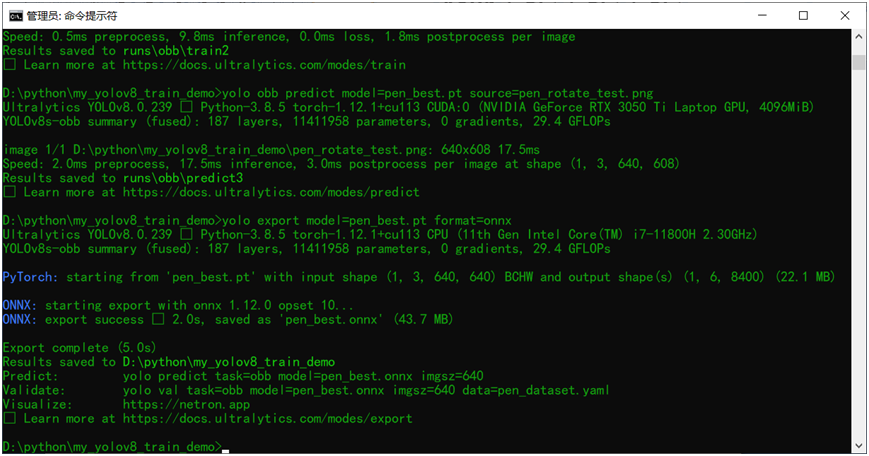
部署推理
转成ONNX格式文件以后,基于OpenVINO-Python部署推理,相关代码如下
class_list = ["pen"]
colors = [(255, 255, 0), (0, 255, 0), (0, 255, 255), (255, 0, 0)]
ie = Core()
for device in ie.available_devices:
print(device)
# Read IR
model = ie.read_model(model="pen_best.onnx")
compiled_model = ie.compile_model(model=model, device_name="CPU")
output_layer = compiled_model.output(0)
## xywhr
frame = cv.imread("D:/python/my_yolov8_train_demo/four_pen.jpg")
bgr = format_yolov8(frame)
img_h, img_w, img_c = bgr.shape
start = time.time()
image = cv.dnn.blobFromImage(bgr, 1 / 255.0, (640, 640), swapRB=True, crop=False)
res = compiled_model([image])[output_layer] # 1x25x8400
rows = np.squeeze(res, 0).T
boxes, confidences, angles, class_ids = post_process(rows)
indexes = cv.dnn.NMSBoxes(boxes, confidences, 0.25, 0.45)
M = np.zeros((2, 3), dtype=np.float32)
for index in indexes:
box = boxes[index]
d1 = -angles[index]
color = colors[int(class_ids[index]) % len(colors)]
pts = [(box[0], box[1]), (box[0]+box[2], box[1]), (box[0]+box[2], box[1]+box[3]), (box[0], box[1]+box[3])]
rrt_pts = get_rotate_point(pts, M, d1, box)
cv.drawContours(frame, [np.asarray(rrt_pts).astype(np.int32)], 0, (255, 0, 255), 2)
cv.putText(frame, class_list[class_ids[index]], (int(box[0]+box[2]/2), int(box[1]+box[3]/2)), cv.FONT_HERSHEY_SIMPLEX, 1.0, (0, 0, 255), 2)
end = time.time()
inf_end = end - start
fps = 1 / inf_end
fps_label = "FPS: %.2f" % fps
cv.putText(frame, fps_label, (20, 45), cv.FONT_HERSHEY_SIMPLEX, 1, (0, 0, 255), 2)
cv.imshow("YOLOv8-OBB Rotate Object Detection", frame)
cv.imwrite("D:/pen_result.jpg", frame)
cv.waitKey(0)
cv.destroyAllWindows()
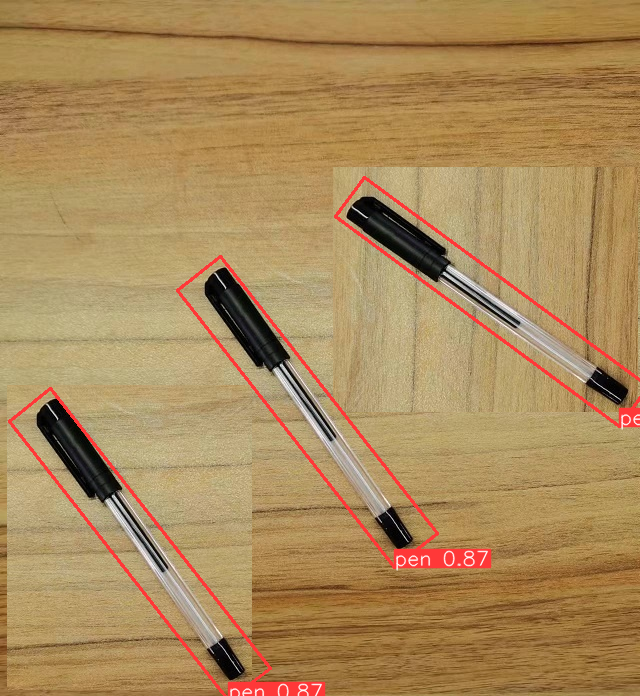
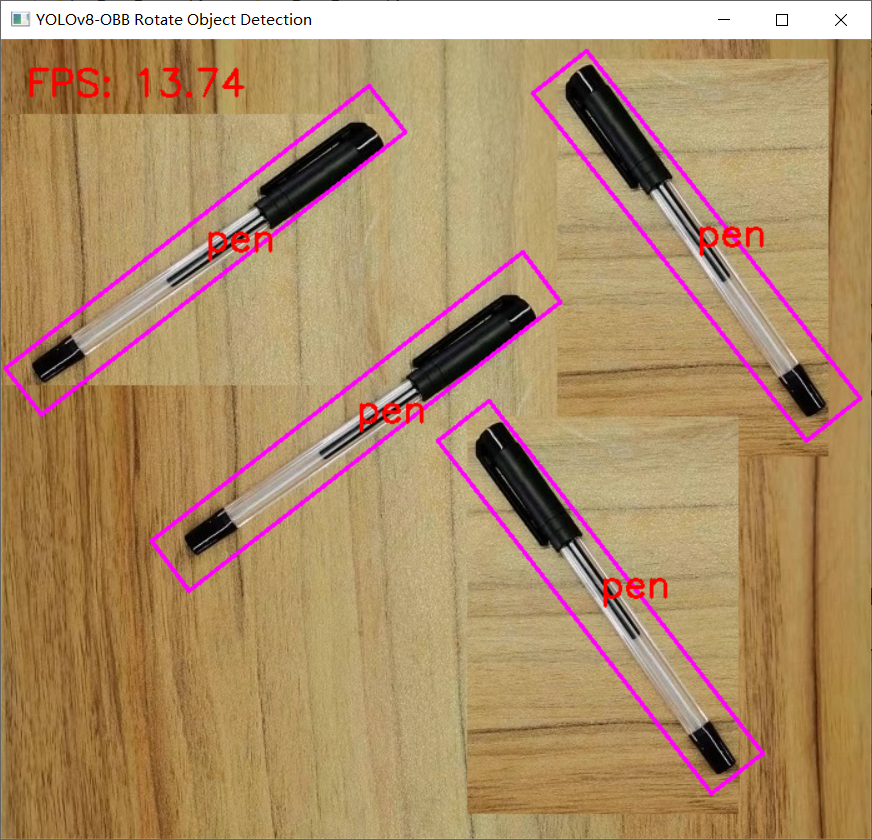