点击下方卡片,关注“小白玩转Python”公众号
在技术快速发展的时代,利用深度学习进行人员识别和追踪彻底改变了监控和安全系统。
深度学习基础知识
要理解如何使用深度学习进行人物识别和跟踪,掌握深度学习的基础知识至关重要。深度学习是机器学习的一个子集,它采用人工神经网络,可以自动从大量数据中学习模式和表示。卷积神经网络 (CNN) 和循环神经网络 (RNN) 是许多用于此目的的深度学习模型的支柱。
物体检测是识别图像或视频流中人物的关键步骤。深度学习模型(例如单次多框检测器 (SSD) 和仅看一次 (YOLO))处于实时物体检测的前沿。这些模型可以以惊人的准确度定位和分类图像或视频帧中的个人。
人脸识别是人脸识别的一个子领域,由于深度学习,人脸识别取得了重大进展。深度神经网络(包括 FaceNet 和 VGGFace)使得根据面部特征准确识别个人成为可能。这些系统广泛用于访问控制、执法和身份验证目的。class FaceNet:
def __init__(
self,
detector: object,
onnx_model_path: str = "assets/models/facenet512_weights.onnx",
anchors: typing.Union[dict] = data,
force_cpu: bool = False,
threshold: float = 0.5,
color: tuple = (255, 255, 255),
thickness: int = 2,
) -> None:
if not stow.exists(onnx_model_path):
raise Exception(f"Model doesn't exists in {onnx_model_path}")
self.detector = detector
self.threshold = threshold
self.color = color
self.thickness = thickness
providers = ['CUDAExecutionProvider', 'CPUExecutionProvider']
providers = providers if ort.get_device() == "GPU" and not force_cpu else providers[::-1]
self.ort_sess = ort.InferenceSession(onnx_model_path, providers=providers)
self.input_shape = self.ort_sess._inputs_meta[0].shape[1:3]
self.anchors = self.load_anchors(anchors) if isinstance(anchors, str) else anchors
def normalize(self, img: np.ndarray) -> np.ndarray:
mean, std = img.mean(), img.std()
return (img - mean) / std
def l2_normalize(self, x: np.ndarray, axis: int = -1, epsilon: float = 1e-10) -> np.ndarray:
output = x / np.sqrt(np.maximum(np.sum(np.square(x), axis=axis, keepdims=True), epsilon))
return output
def detect_save_faces(self, image: np.ndarray, output_dir: str = "faces"):
face_crops = [image[t:b, l:r] for t, l, b, r in self.detector(image, return_tlbr=True)]
if face_crops == []:
return False
stow.mkdir(output_dir)
for index, crop in enumerate(face_crops):
output_path = stow.join(output_dir, f"face_{str(index)}.png")
cv2.imwrite(output_path, crop)
print("Crop saved to:", output_path)
self.anchors = self.load_anchors(output_dir)
return True
def load_anchors(self, faces_path: str):
anchors = {}
if not stow.exists(faces_path):
return {}
for face_path in stow.ls(faces_path):
anchors[stow.basename(face_path)] = self.encode(cv2.imread(face_path.path))
return anchors
def encode(self,
face_image: np.ndarray) -> np.ndarray:
face = self.normalize(face_image)
face = cv2.resize(face, self.input_shape).astype(np.float32)
encode = self.ort_sess.run(None, {self.ort_sess._inputs_meta[0].name: np.expand_dims(face, axis=0)})[0][0]
normalized_encode = self.l2_normalize(encode)
return normalized_encode
def l1_distance(self, a: np.ndarray, b: typing.Union[np.ndarray, list]) -> np.ndarray:
if isinstance(a, list):
a = np.array(a)
if isinstance(b, list):
b = np.array(b)
return np.sum(np.abs(a - b))
def cosine_distance(self, a: np.ndarray, b: typing.Union[np.ndarray, list]) -> np.ndarray:
if isinstance(a, list):
a = np.array(a)
if isinstance(b, list):
b = np.array(b)
return np.dot(a, b.T) / (np.linalg.norm(a) * np.linalg.norm(b))
def draw(self, image: np.ndarray, face_crops: dict):
for value in face_crops.values():
t, l, b, r = value["tlbr"]
cv2.rectangle(image, (l, t), (r, b), self.color, self.thickness)
name = stow.name(value['name'])
name = name.rsplit('_')[0]
cv2.putText(image, name, (l, t - 10), cv2.FONT_HERSHEY_SIMPLEX, 0.9, self.color, self.thickness)
return image
def __call__(self, frame: np.ndarray,face_frame=False) -> np.ndarray:
names = None
if not face_frame:
face_crops = {index: {"name": "", "tlbr": tlbr} for index, tlbr in enumerate(self.detector(frame, return_tlbr=True))}
for key, value in face_crops.items():
t, l, b, r = value["tlbr"]
face_encoding = self.encode(frame[t:b, l:r])
distances = self.cosine_distance(face_encoding, list(self.anchors.values()))
if np.max(distances) > self.threshold:
face_crops[key]["name"] = list(self.anchors.keys())[np.argmax(distances)]
names = face_crops[key]["name"]
names = names.rsplit('_')[0]
print(names,np.max(distances))
else:
face_encoding = self.encode(frame)
distances = self.cosine_distance(face_encoding, list(self.anchors.values()))
if np.max(distances) > self.threshold:
names = list(self.anchors.keys())[np.argmax(distances)].rsplit('_')[0]
print(names,np.max(distances))
return names
人员跟踪涉及监控和跟踪个人在场景中或视频帧中移动的情况。DeepSORT(单对象跟踪深度学习)和 SORT(简单在线实时跟踪)是利用深度学习来提高准确性和稳健性的跟踪算法的示例。方法
- 物体检测系统处理帧并从场景中提取人物。对于每个人,都会裁剪帧的一个子区域以进行详细处理。
- 每个人的区域都经过面部检测算法的处理,从身体中提取出一个人的脸部。
- 每张脸都经过人脸识别系统扫描,系统会将当前人脸与数据库中存储的人脸进行比较。如果识别出人脸,则返回姓名,否则返回“未定义”。
将识别与唯一的跟踪 ID 关联起来是人物识别和跟踪系统中一种常见且有效的方法,尤其是在个人可能进出视野或暂时遮挡脸部的情况下。此方法可确保即使在给定帧中某人的脸部暂时被遮挡或不再可见,系统仍可以根据其分配的跟踪 ID 识别他们。其工作原理如下:- 分配跟踪 ID:系统为检测到的人分配唯一的跟踪 ID。此 ID 与他们的面部特征和其他相关信息相关联。
- 持续跟踪:随着视频流或帧的进展,跟踪算法会持续监控个人的动作和外观。即使一个人的脸暂时被遮挡或不再可见,系统仍会根据其唯一的跟踪 ID 跟踪他们的动作。
- 重新识别:当人的脸部变得不可见时,系统可以通过匹配其当前跟踪 ID 来重新识别他们。即使在具有挑战性的场景中,也可以跨不同帧无缝跟踪个人。
if bool(name):
to_remove = []
for key, value in id_face_dictionary.items():
if value == name:
if id != key:
to_remove.append(key)
loggers["recognition"].info(f"{name} already in dict. ID: {id}")
for k in to_remove:
id_face_dictionary.pop(k)
id_face_dictionary[id] = name
loggers["recognition"].info(f"Added {name} to key {id}")
通过使用跟踪 ID,系统可以在整个视频或帧序列中为每个人保持一致的身份,确保即使脸部始终不可见也能保持识别。这种方法在包括视频监控在内的各种应用中都很有价值,在这些应用中,持续跟踪和识别对于安全和分析目的至关重要。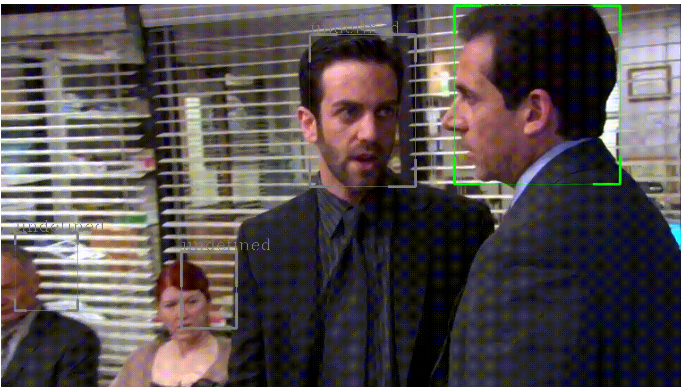
优化实时物体检测和追踪
实时物体检测和跟踪是各种应用(例如监控、自动驾驶和交互系统)中的关键组件。然而,在每帧 30 毫秒 (ms) 的严格时间限制内执行检测和跟踪(相当于每秒 30 帧 (fps) 的帧速率)会带来巨大的计算挑战。为了克服这个问题,我们提出了一种多线程架构,将处理分为三个独立线程:核心、检测器和识别器。每个线程都设计为同时运行,从而减少处理延迟和资源争用。此线程可确保始终处理最新的帧。如果检测器线程处理一帧的时间超过30 毫秒,则核心线程会跳过该线程,从而避免积压并确保实时性能,而无需对帧进行排队。while vid.isOpened():
ret, frame = vid.read()
# out = None
if ret:
if queuepulls == 1:
timer2 = time.time()
# Capture frame-by-frame
# if the input queue *is* empty, give the current frame to
# classify
if inputQueue.empty():
inputQueue.put(frame)
else:
loggers["general"].debug("Skipping frame from face detection")
# if the output queue *is not* empty, grab the detections
if not outputQueue.empty():
out = outputQueue.get()
if out is not None:
queuepulls += 1
for output in out:
bbox_left = int(output[0])
bbox_top = int(output[1])
bbox_w = int(output[2])
bbox_h = int(output[3])
if output.shape[0] == 7:
id = int(output[4])
prev_id = id
else:
id =prev_id
if id in id_face_dictionary:
name = id_face_dictionary[id]
else:
name = "undefined"
color = (255,0,0) # Use your custom color
drawPerson(frame,bbox_left,bbox_top,bbox_w,bbox_h,name,color)
cv2.imshow('frame', frame)
if cv2.waitKey(1) & 0xFF == ord('q'):
vid.release()
cv2.destroyAllWindows()
p.kill()
pRec.kill()
break
该探测器注重速度和准确性,采用优化的算法,能够在 30 毫秒的时间内识别各种物体。def object_detection_(model_path,confidence,inputQueue,outputQueue,recognitionQueue):
global id_face_dictionary
yolov8_detector = YOLO(model_path)
device = torch.device('cuda' if torch.cuda.is_available() else 'cpu')
yolov8_detector.to(device)
loggers['tracking'].info("Detection initialized")
while True:
if not inputQueue.empty():
frame = inputQueue.get()
result = yolov8_detector.track(frame,verbose=
False,conf=confidence,persist=True)[0]
data = result.cpu().numpy().boxes.data
outputQueue.put(data)
if recognitionQueue.empty():
recognitionQueue.put((data,frame))
它还以无限循环的方式运行,检查来自探测器的新数据并立即处理以识别视频帧中的个人或特征。def recognize_algorithm(model_path,recognitionQueue,id_face_dictionary,confidence):
detector = face_detector.FaceDetection()
recog = face_recognition.FaceNet(
detector=detector,
threshold=confidence,
onnx_model_path = model_path)
loggers['recognition'].info("Recognition initialized")
while True:
if not recognitionQueue.empty():
out = recognitionQueue.get()
frame = out[1]
boxes = out[0]
for output in boxes:
bbox_left = int(output[0])
bbox_top = int(output[1])
bbox_w = int(output[2])
bbox_h = int(output[3])
id = int(output[4])
if bbox_w > 0 and bbox_h > 0:
person_frame = frame[bbox_top:bbox_h,bbox_left:bbox_w,:]
start_time = time.time()
name = recog(frame=person_frame,face_frame=True)
loggers['recognition'].debug(f"RECOGNITION - Inference time: {round(time.time()-start_time,2)}")
if bool(name):
to_remove = []
for key, value in id_face_dictionary.items():
if value == name:
if id != key:
to_remove.append(key)
loggers["recognition"].info(f"{name} already in dict. ID: {id}")
for k in to_remove:
id_face_dictionary.pop(k)
#once deleted, we add new key
id_face_dictionary[id] = name
loggers["recognition"].info(f"Added {name} to key {id}")
线程间通信是此架构的基石。它允许异步处理帧,其中每个线程独立检查新帧并处理它们。这种设计确保系统始终在最新的可用帧上工作,从而保持实时性能而不会出现延迟。每个线程通过Python 队列进行通信,并采用同步机制来防止竞争条件和数据损坏。 inputQueue = Queue(maxsize=1)
outputQueue = Queue(maxsize=1)
recognitionQueue = Queue()
p = Process(target=object_detection_, args=(model_path,detection_confidence,inputQueue, outputQueue,recognitionQueue,))
p.daemon = True
p.start()
pRec = Process(target=recognize_algorithm, args=(recognition_model_path,recognitionQueue,id_face_dictionary,recognition_confidence,))
pRec.daemon = True
pRec.start()
系统规格
该应用程序旨在无缝运行在 Python 上,让不同操作系统的广大用户都能使用它。它的跨平台兼容性确保它可以在 Windows、macOS 和各种 Linux 发行版等流行操作系统上使用。虽然该应用程序在操作系统支持方面用途广泛,但需要注意的是,为了获得最佳实时性能,强烈建议使用 GPU(图形处理单元),尤其是在使用资源密集型深度学习模型时。GPU 可以显著加速这些模型的执行,实现更快的处理速度并增强应用程序高效执行实时任务的能力。