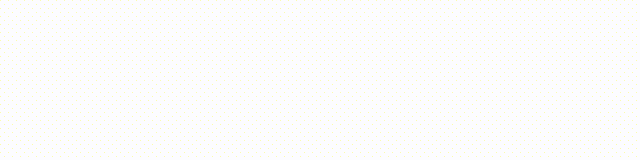
活动名称:
机器学习贝叶斯力场及量化不确定性的分子动力学模拟
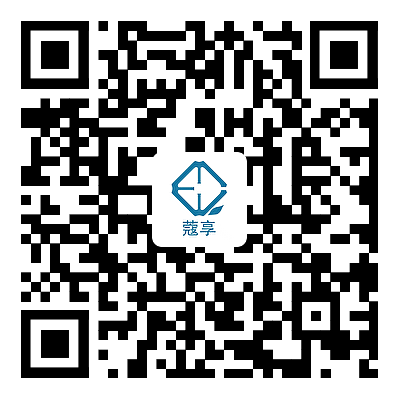
| 蔻享学术直播间
|
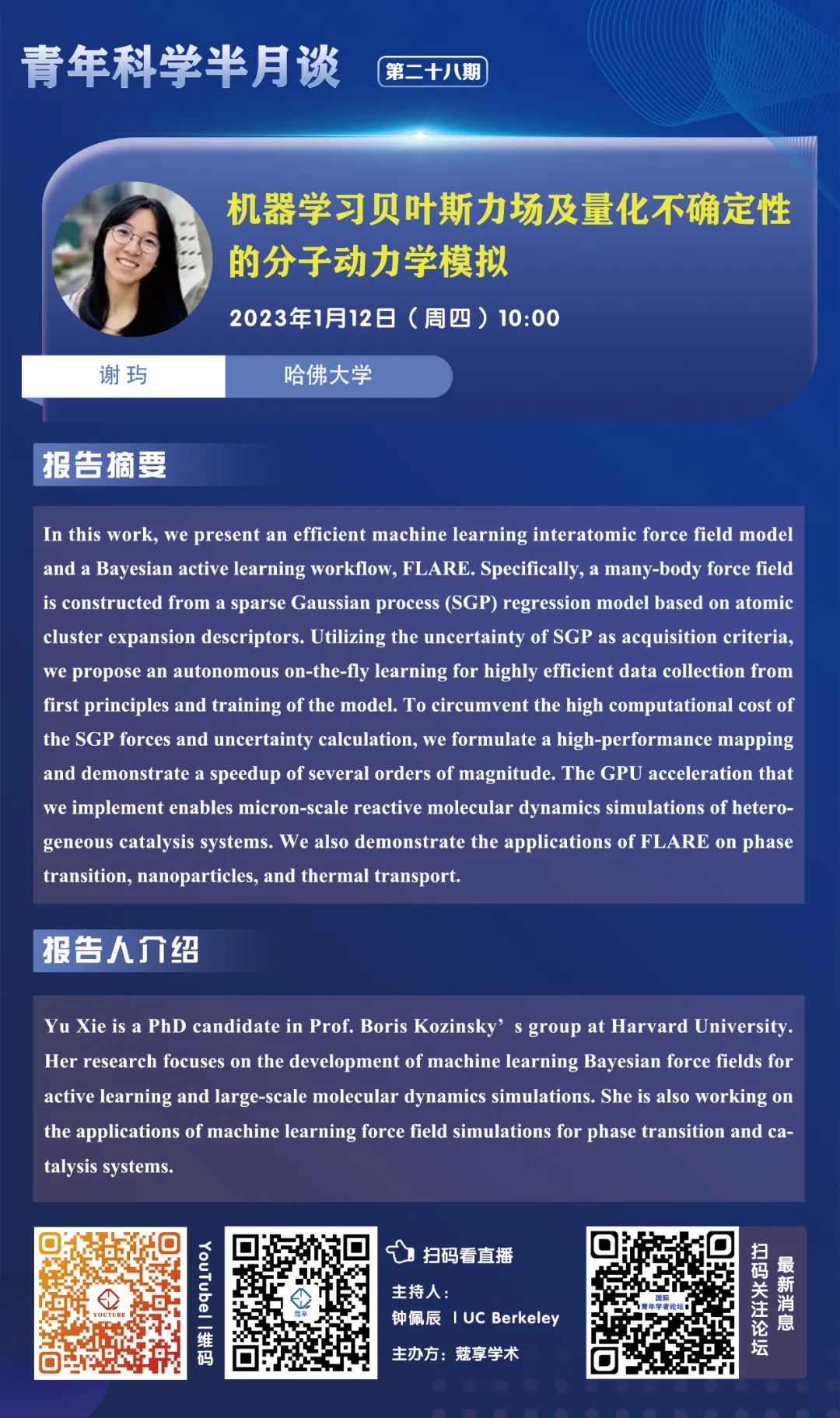
Yu Xie is a PhD candidate in Prof. Boris Kozinsky’s group at Harvard University. Her research focuses on the development of machine learning Bayesian force fields for active learning and large-scale molecular dynamics simulations. She is also working on the applications of machine learning force field simulations for phase transition and catalysis systems.
In this work, we present an efficient machine learning interatomic force field model and a Bayesian active learning workflow, FLARE. Specifically, a many-body force field is constructed from a sparse Gaussian process (SGP) regression model based on atomic cluster expansion descriptors. Utilizing the uncertainty of SGP as acquisition criteria, we propose an autonomous on-the-fly learning for highly efficient data collection from first principles and training of the model. To circumvent the high computational cost of the SGP forces and uncertainty calculation, we formulate a high-performance mapping and demonstrate a speedup of several orders of magnitude. The GPU acceleration that we implement enables micron-scale reactive molecular dynamics simulations of heterogeneous catalysis systems. We also demonstrate the applications of FLARE on phase transition, nanoparticles, and thermal transport.
蔻享学术平台,国内领先的一站式科学资源共享平台,依托国内外一流科研院所、高等院校和企业的科研力量,聚焦前沿科学,以优化科研创新环境、传播和服务科学、促进学科交叉融合为宗旨,打造优质学术资源的共享数据平台。
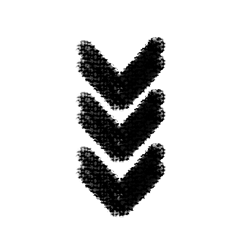
点击阅读原文,查看更多精彩报告!