官网:https://auto.gluon.ai/
安装:https://auto.gluon.ai/stable/install.html
Quick Demo:基础使用
https://auto.gluon.ai/stable/tutorials/tabular_prediction/tabular-quickstart.html
我们先来展示一个简单结构化的案例,通过使用TabularPredictor
和TabularDataset
。
from autogluon.tabular import TabularDataset, TabularPredictor
# 通过路径加载数据
train_data = TabularDataset('https://autogluon.s3.amazonaws.com/datasets/Inc/train.csv')
subsample_size = 500
train_data = train_data.sample(n=subsample_size, random_state=0)
# 训练多个模型
predictor = TabularPredictor(label='class').fit(train_data)
# 模型预测
y_pred = predictor.predict(test_data_nolab)
print("Predictions: \n", y_pred)
perf = predictor.evaluate_predictions(y_true=y_test, y_pred=y_pred, auxiliary_metrics=True)
在这个例子中主要的时间会运行fit()
,AutoGluon
会分析标签的类型确定是一个二分类任务,然后确定具体的评价指标,接下来是推测每一个特征的类型。
AutoGluon
自动选择数据的随机训练/验证拆分。用于验证的数据与训练数据分开,用于确定产生最佳结果的模型和超参数值。AutoGluon
不仅仅是一个模型,而是训练多个模型并将它们集成在一起以确保卓越的预测性能。
AutoGluon
会尝试拟合各种类型的模型,包括神经网络和树集成。每种类型的模型都有各种超参数,传统上,用户必须指定这些超参数。AutoGluon
自动迭代地测试超参数的值,以在验证数据上产生最佳性能。
predictor.leaderboard(test_data, silent=True)
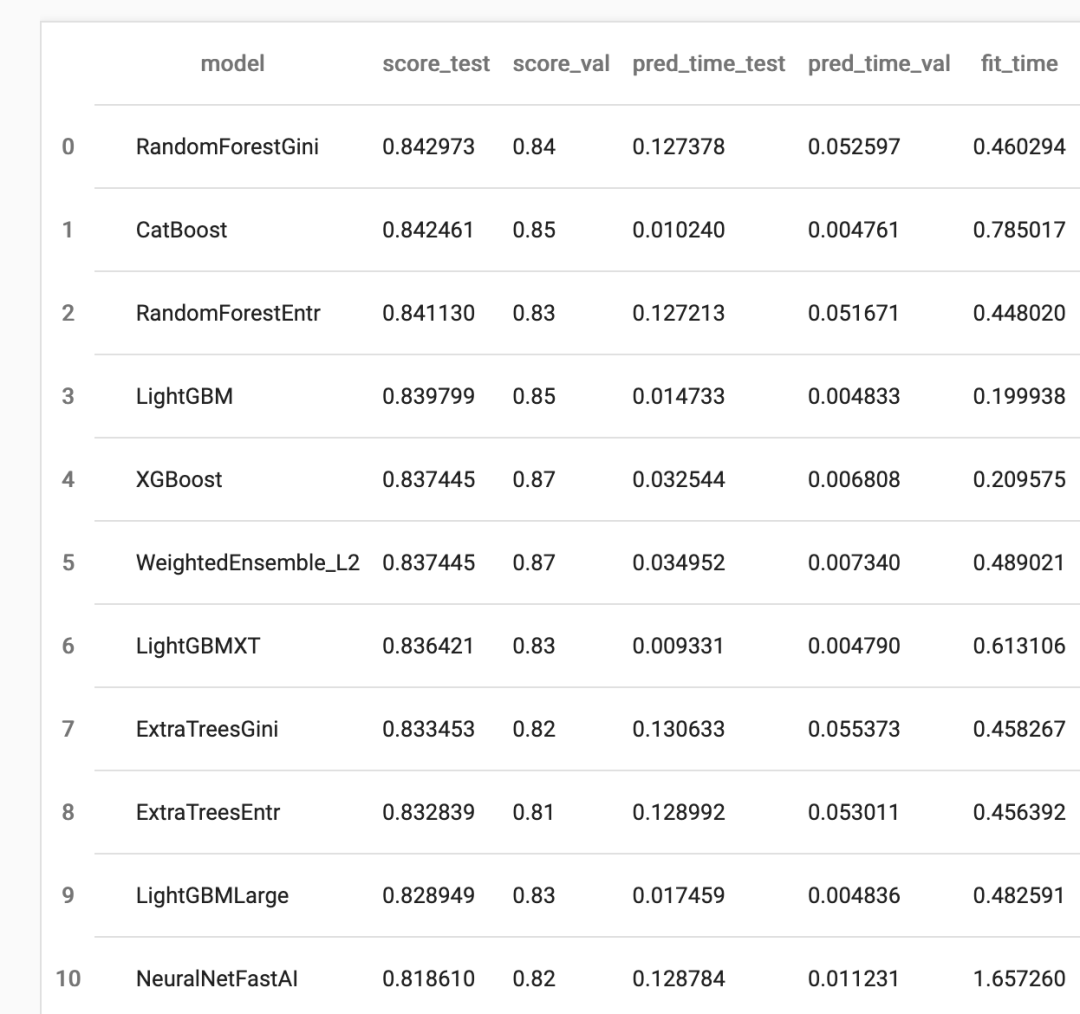
Quick Demo:进阶使用
https://auto.gluon.ai/stable/tutorials/tabular_prediction/tabular-indepth.html
在AutoGluon
可以指定超参数进行调整,可以指定单个固定值,或在超参数优化期间要考虑的值的搜索空间。
import autogluon.core as ag
nn_options = { # specifies non-default hyperparameter values for neural network models
'num_epochs': 10, # number of training epochs (controls training time of NN models)
'learning_rate': ag.space.Real(
1e-4, 1e-2, default=5e-4, log=True), # learning rate used in training (real-valued hyperparameter searched on log-scale)
'activation': ag.space.Categorical('relu', 'softrelu', 'tanh'), # activation function used in NN (categorical hyperparameter, default = first entry)
'dropout_prob': ag.space.Real(0.0, 0.5, default=0.1), # dropout probability (real-valued hyperparameter)
}
gbm_options = { # specifies non-default hyperparameter values for lightGBM gradient boosted trees
'num_boost_round': 100, # number of boosting rounds (controls training time of GBM models)
'num_leaves': ag.space.Int(lower=26, upper=66, default=36), # number of leaves in trees (integer hyperparameter)
}
hyperparameters = { # hyperparameters of each model type
'GBM': gbm_options,
'NN_TORCH': nn_options, # NOTE: comment this line out if you get errors on Mac OSX
} # When these keys are missing from hyperparameters dict, no models of that type are trained
time_limit = 2*60 # train various models for ~2 min
num_trials = 5 # try at most 5 different hyperparameter configurations for each type of model
search_strategy = 'auto' # to tune hyperparameters using random search routine with a local scheduler
hyperparameter_tune_kwargs = { # HPO is not performed unless hyperparameter_tune_kwargs is specified
'num_trials': num_trials,
'scheduler' : 'local',
'searcher': search_strategy,
}
predictor = TabularPredictor(label=label, eval_metric=metric).fit(
train_data, tuning_data=val_data, time_limit=time_limit,
hyperparameters=hyperparameters, hyperparameter_tune_kwargs=hyperparameter_tune_kwargs,
)
除了使用超参数调整之外,另外两种提高预测性能的方法是bagging 和 stack,但这会增加训练时间和内存/磁盘使用量。
predictor = TabularPredictor(label=label, eval_metric=metric).fit(train_data,
num_bag_folds=5, num_bag_sets=1, num_stack_levels=1,
hyperparameters = {'NN_TORCH': {'num_epochs': 2}, 'GBM': {'num_boost_round': 20}}, # last argument is just for quick demo here, omit it in real applications
)
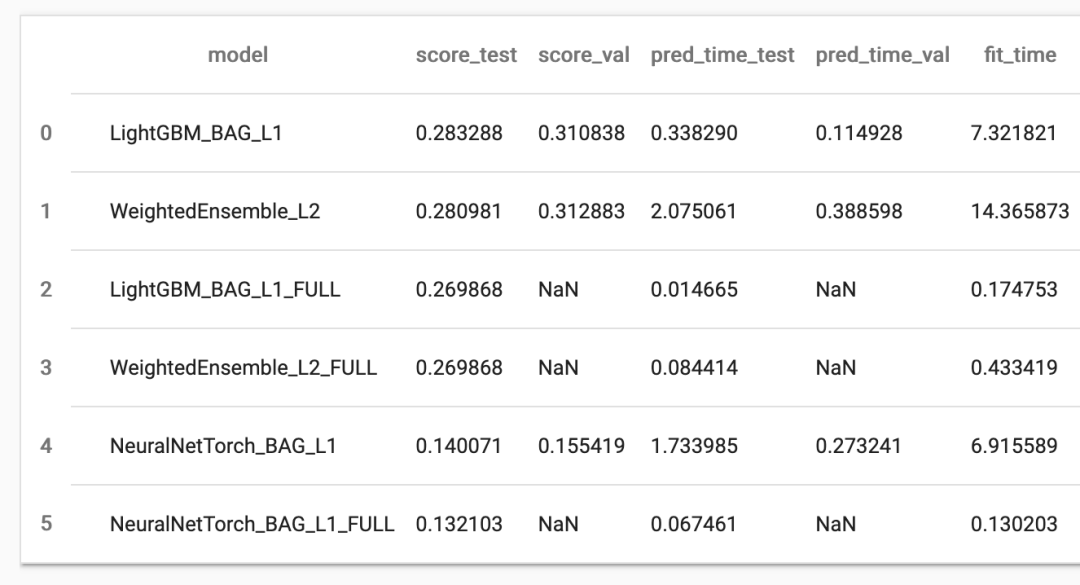
AutoGluon 结构化模型
模型名称 | 文档地址 |
---|
LGBModel | LightGBM model: https://lightgbm.readthedocs.io/en/latest/ |
CatBoostModel | CatBoost model: https://catboost.ai/ |
XGBoostModel | XGBoost model: https://xgboost.readthedocs.io/en/latest/ |
RFModel | Random Forest model (scikit-learn): https://scikit-learn.org/stable/modules/generated/sklearn.ensemble.RandomForestClassifier.html |
XTModel |
Extra Trees model (scikit-learn): https://scikit-learn.org/stable/modules/generated/sklearn.ensemble.ExtraTreesClassifier.html#sklearn.ensemble.ExtraTreesClassifier |
KNNModel | KNearestNeighbors model (scikit-learn): https://scikit-learn.org/stable/modules/generated/sklearn.neighbors.KNeighborsClassifier.html |
LinearModel | Linear model (scikit-learn): https://scikit-learn.org/stable/modules/generated/sklearn.linear_model.LogisticRegression.html |
TabularNeuralNetTorchModel | PyTorch neural network models for classification/regression with tabular data. |
TabularNeuralNetMxnetModel | Class for neural network models that operate on tabular data. |
NNFastAiTabularModel | Class for fastai v1 neural network models that operate on tabular data. |
VowpalWabbitModel | VowpalWabbit Model: https://vowpalwabbit.org/ |